Much data in the engineering world exists in the form of paper documents and drawings. Technically, these are considered unstructured data because extracting content from such drawings using traditional programs is highly resource intensive. However, artificial intelligence (AI) systems can be trained to recognize visual content in drawings and provide a simplified context. The complete paper emphasizes the use of AI in processing a scanned drawing and redrawing it on a digital platform.
×
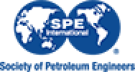
Continue Reading with SPE Membership
SPE Members: Please sign in at the top of the page for access to this member-exclusive content. If you are not a member and you find JPT content valuable, we encourage you to become a part of the SPE member community to gain full access.