Artificial intelligence (AI) has captivated the imagination of science-fiction movie audiences for many years and has been used in the upstream oil and gas industry for more than a decade (Mohaghegh 2005, 2011).
But few industries evolve more quickly than those from Silicon Valley, and it accordingly follows that the technology has grown and changed considerably since this discussion began.
The oil and gas industry, therefore, is at a point where it would be prudent to take stock of what has been achieved with AI in the sector, to provide a sober assessment of what has delivered value and what has not among the myriad implementations made so far, and to figure out how best to leverage this technology in the future in light of these learnings.
When one looks at the long arc of AI in the oil and gas industry, a few important truths emerge. First among these is the fact that not all AI is the same. There is a spectrum of technological sophistication. Hollywood and the media have always been fascinated by the idea of artificial superintelligence and general intelligence systems capable of mimicking the actions and behaviors of real people.
Those kinds of systems would have the ability to learn, perceive, understand, and function in human-like ways (Joshi 2019). As alluring as these types of AI are, however, they bear little resemblance to what actually has been delivered to the upstream industry.
Instead, we mostly have seen much less ambitious “narrow AI” applications that very capably handle a specific task, such as quickly digesting thousands of pages of historical reports (Kimbleton and Matson 2018), detecting potential failures in progressive cavity pumps (Jacobs 2018), predicting oil and gas exports (Windarto et al. 2017), offering improvements for reservoir models (Mohaghegh 2011), or estimating oil-recovery factors (Mahmoud et al. 2019).
But let’s face it: As impressive and commendable as these applications have been, they fall far short of the ambitious vision of highly autonomous systems that are capable of thinking about things outside of the narrow range of tasks explicitly handed to them. What is more, many of these narrow AI applications have tended to be modified versions of fairly generic solutions that were originally designed for other industries and that were then usefully extended to the oil and gas industry with a modest amount of tailoring. In other words, relatively little AI has been occurring in a way that had the oil and gas sector in mind from the outset.
The second important truth is that human judgment still matters. What some technology vendors have referred to as “augmented intelligence” (Kimbleton and Matson 2018), whereby AI supplements human judgment rather than supplants it, is not merely an alternative way of approaching AI; rather, it is coming into focus that this is probably the most sensible way forward for this technology.
The industry’s early forays into AI have confirmed something that experienced engineers and managers in the sector have long understood: Experience counts. Left to their own devices, AI algorithms sometimes get too preoccupied with the wrong relationships within the data, and it is indispensable in those moments to have the benefit of a seasoned veteran to teach the algorithm what is actually important in the physical system.
We, therefore, will need to continue to safeguard and actively manage the deep technical expertise within the oil and gas industry’s people because that know-how will prove to be a necessary foundation for taking AI to the next level in the sector. Combining the petroleum engineering community’s deep understanding of the industry’s technical dimensions—for example, the complex physics behind subsurface phenomena—with the predictive power of AI will yield far better results than either physics or pure AI could achieve on their own.
The third emerging truth about AI in the oil and gas industry pertains to where and how the future of the sector will unfold. Evolutionary adjustments from older technological paradigms to newer ones sometimes carry with them pronounced geographical shifts in where industries are physically located. The US car industry is a timely example of this.
Detroit has long been the epicenter of American automotive innovation since the time of Henry Ford, but the explosive growth of digital technologies and software in modern automotive designs has made the Motor City’s once dominant position as a technology hub in the vehicle industry seem less certain today.
Tesla, a company that has played a leading role in the application of next-generation automotive technologies such as electric propulsion systems, batteries, and self-driving capabilities, became the most valuable car company in the world a few months ago (BBC 2020); and its headquarters are tellingly located near Silicon Valley, thousands of miles away from Detroit. As the upstream oil and gas industry becomes increasingly digital in nature, might such a shift be in the cards for this sector, too?
What we have seen from AI’s trajectory so far suggests that this kind of conspicuous pivot to Silicon Valley probably will not happen in the energy industry. There is no denying that, as the oil and gas industry becomes more and more driven by digital innovations, Silicon Valley will continue to play an important role in the sector’s future.
Some high-profile technology companies have announced that they are backing away from making customized AI tools for the oil and gas industry (Shead 2020), but the know-how behind this technology is sufficiently widespread that this gesture will probably not stop this technology from continuing to take root in the industry.
AI will continue to make inroads in the sector and will gradually move beyond the narrow AI applications that have been delivered thus far.
Silicon Valley cannot do this alone, however. As noted earlier, digital companies will need the deep experience and human know-how that the oil and gas industry has cultivated over many years in Houston and other energy technology hubs like it.
A few Houston-area companies may be reaching out to work more closely with California-based firms, but, at the same time, Silicon Valley startups are doing the same thing in the opposite direction. These two communities will need each other to take AI to the next level together in the upstream oil and gas industry.
Science-fiction movie enthusiasts and admirers of AI could be forgiven for being less than excited about the progress that has been made throughout the early days of this technology in the oil and gas sector.
Several impressive applications and implementations have happened, but clearly nothing like the higher levels of intelligence that Hollywood hinted we would be enjoying by now. However, we have seen this movie before, too. As the futurist Roy Amara once famously observed, “We tend to overestimate the effect of a technology in the short run and underestimate the effect in the long run.”
The story of AI in the oil and gas industry has barely begun, and the sector has a long and well-documented history of slowly warming up to new technologies before embracing them enthusiastically.
That enthusiastic inflection point has now arrived. We should all brace ourselves for a very quick increase in the rate and amount of AI-related innovation happening within the industry.
References
BBC (2020). Tesla Overtakes Toyota To Become World’s Most Valuable Carmaker.
Jacobs, T. (2018). Shell Picks a Digital Platform To Build Its AI Future Upon. J Pet Technol, 70 (12), 43–44.
Joshi, N. (2019).7 Types of Artificial Intelligence.
Kimbleton, S. and Matson, J. (2018). Guest Editorial: Cognitive Computing: Augmenting Human Intelligence To Improve Oil and Gas Outcomes. J Pet Technol, 70 (04), 14–15.
Mahmoud, A.A. and Elkatatny, S., et al. (2019). Estimation of Oil Recovery Factor for Water Drive Sandy Reservoirs Through Applications of Artificial Intelligence. Energies, 12 (19), 3671.
Mohaghegh, S.D. (2005). Recent Developments in Application of Artificial Intelligence in Petroleum Engineering. J Pet Technol, 57 (04), 86–91.
Mohaghegh, S.D. (2011). Reservoir Simulation and Modeling Based on Artificial Intelligence and Data Mining (AI&DM). J Nat Gas Eng, 3 (6), 697–705.
Shead, S. (2020). Google Plans to Stop Making AI Tools for Oil and Gas Firms.
Windarto, A.P. and Dewi, L.S. et al. (2017). Implementation of Artificial Intelligence in Predicting the Value of Indonesian Oil and Gas Exports With BP Algorithm. Int J Recent Trends Eng Res, 3 (10), 1–12.
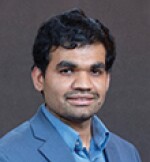
Manas Pathak is the global artificial intelligence (AI) lead for oil and gas at Intel. He holds a PhD degree in chemical engineering from the University of Utah and an integrated master’s in applied geology from the Indian Institute of Technology, Indian School of Mines, Dhanbad. In his PhD research, he used machine learning to optimize oil production. He is an author of multiple journal papers in oil and gas, and a well-accepted speaker at SPE, AAPG, EAGE, and other energy-related conferences. He also serves as a technical reviewer for Elsevier and Nature.
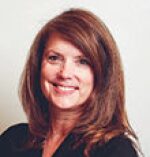
Tonya Cosby is Intel’s global enterprise oil and gas account manager. In this role, she articulates Intel’s data-centric strategies to help customers manage the unending growth of data. Her role includes projects/solutions involving cloud computing, Internet of things, blockchain, artificial intelligence, and automated vehicles. She holds a BS degree in business/managerial economics from Oklahoma State University.
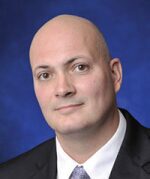
Robert K. Perrons, SPE, is a professor at the Queensland University of Technology in Brisbane, Australia. Before joining the university in 2011, he worked in a variety of roles for Shell International’s Exploration and Production division. He started his career in the strategy and economics team, worked for several years as a production engineer in overseas operations (offshore and onshore), and served as the company’s executive coordinator of global R&D. Perrons holds a BEng degree in mechanical engineering from McMaster University, a master’s degree in technology and policy from the Massachusetts Institute of Technology, and a PhD degree in engineering from the University of Cambridge, where he was a Gates Cambridge Scholar. He is a member of the United Nations’ Resources and Energy Expert Group and was named as a Fulbright Scholar in 2020. For more information and contact details, please visit www.perrons.net.