The Internet of Things (IoT), along with advances in sensor technology, data analytics, and artificial intelligence (AI), has paved the way for significant efficiency and productivity gains in the oil and gas industry. One application in particular, electrical submersible pumps (ESPs), has benefited from these advances. The complete paper explores an AI-based application that enables operators to preempt costly ESP failures while optimizing production.
AI and Machine Learning
Cloud-based platforms can provide offshore operators with access to advanced analytics software featuring AI algorithms that analyze incoming data for anomalies that could signal trouble ahead in the monitored equipment. This type of AI capability is often referred to as machine learning (ML). ML uses statistical analysis to infer the probability of a hypothesized event, combining this capability with neural network models to learn the relational behavior of a system and its components.
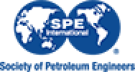