In recent years, artificial intelligence (AI) -based detection algorithms increasingly have been proposed in the literature for diagnosis of torsional stick/slip vibration. One drawback of AI methods, however, is their limited applicability to wells in the same field with similar geological formations and bottomhole assemblies (BHAs). The complete paper presents trained and tested machine-learning (ML) models capable of predicting the stick/slip severity index (SSI) using sequences of surface measurements and physical features. The paper also proposes real-time auto-updated stability heat maps to help drillers choose optimal drilling parameters to avoid stick/slip vibrations.
Modeling Approach
In this section of the complete paper, the authors introduce a regression model aimed at predicting a continuous nondiscrete SSI. The model takes as input time-series data of drilling surface measurements, including surface torque, surface weight on bit (WOB), rate of penetration (ROP), flow rate, and total rotation speed. These measurements were selected based on their relevance for addressing the stick/slip problem and their widespread availability in most wells.
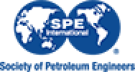