The complete paper discusses optimization of a development plan involving low-salinity water injection (LSWI). This methodology combines compositional simulation and a mathematical optimization tool that uses artificial intelligence to maximize the net present value (NPV) of the project under evaluation. The adapted methodology allowed an optimal development plan, considering the uncertainty associated in the reservoir, by use of multiple geostatistical realizations and simultaneous history-matched models.
LSWI
LSWI is an enhanced-oil-recovery technique in which the salinity of the injected water is controlled with the objective of increasing the recovery factor. Of the mechanisms proposed for LSWI, wettability alteration is the most widely accepted for describing the increase of the recovery factor. The wettability change in reservoirs with the presence of sandstones mainly is attributed to the multionic exchange between the injected fluid and the clay surface, as is the double layer expansion.
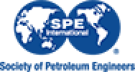