Throughout the present decade, the oil and gas industry is experiencing and enduring digital transformation, marked by continuous evolution. In the pursuit of increased efficiency, companies have embraced cutting-edge technologies in artificial intelligence and big data, effectively automating their existing work flows.
Having undergone extensive exploration and refinement, the debate between physics-based models and data-driven models has now found a harmonious middle ground. This convergence has paved the way for significant advancements in the realms of transfer learning and machine-learning-assisted simulation, opening up new avenues for research and practical application.
The paramount objective of data scientists in the oil and gas industry is to decipher hidden patterns within the data and subsequently transform these insights into domain knowledge, which, in turn, guides operation and facilitates educated decision-making. Hence, despite the thrilling advancements in deep learning and large language models, the upstream industry continues to extensively use traditional machine-learning models such as tree-based algorithms for processing structured data sets because of their strong interpretability.
Another prominent trend involves the widespread development and application of no-code or low-code machine-learning platforms, empowering citizen data scientists to efficiently leverage machine-learning models.
As we press forward, we are confronted with a series of challenges, including the following:
- Coping with an abundance of data with low quality that need significant effort in manipulation and quality control
- Contending with the relatively high cost of data acquisition, which curtails the full exploitation of data-hungry models
- Encountering a scarcity of successful proof-of-concept projects that make it to production
- Lacking interpretability of complex but powerful models, hindering our ability to deliver tangible insight to subject-matter experts and management
The selected list of papers shares some interesting examples to showcase the value added by close collaboration between data scientists and subject-matter experts and how the power of the digital revolution actively revitalizes our industry.
This Month’s Technical Papers
Study Reviews Eagle Ford Parent-Well Frac-Hit Analysis, Prediction, and Mitigation
Data Analytics Enables Reconstruction of Missing Segments in Well Data History
Deep Learning Enhances Transfer Learning for Tight-Oil Production Prediction
Recommended Additional Reading
SPE 204712 Data-Driven Solution for Enhancing Workover Intervention Activities by Saniya Karnik, SLB, et al.
SPE 211363 Generation of a Regional Fluid Database for Gas Condensate Assets in a Thrusted Carbonate Environment of the Northern Emirates by Harshil Saradva, Sharjah National Oil Corporation, et al.
SPE 211719 Machine-Learning-Assisted Well-Log Data Quality Control and Preprocessing Lab by Nader Gerges, ADNOC, et al.
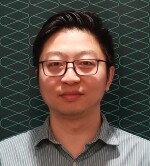
Junjie Yang, SPE, is a senior simulation engineer with Chevron. He has 10 years of experience in the oil and gas industry. Yang’s specialties include analytical and numerical reservoir modeling, hydraulic-fracture modeling, and optimization. He is also skilled in data analytics as well as integrated statistical analysis in production forecasting, rejuvenation candidate selection, and field development strategy optimization. Yang has been leading and contributed to multidisciplinary consulting projects from major conventional and unconventional plays across North America, including superhot rock geothermal asset stimulation and development. He holds a PhD degree in petroleum and natural gas engineering from The Pennsylvania State University.