A physics-assisted deep-learning model is presented to facilitate transfer learning in unconventional reservoirs by integrating the complementary strengths of physics-based and data-driven predictive models. The developed model uses a deep-learning architecture to map formation properties to their corresponding production responses using a low-dimensional feature space representation. The results indicate that physics-based simulated data can facilitate production predictions when out-of-range (unseen) input parameters have to extrapolate from data and that transferring the weights learned from the source field to the target field can add valuable information to enhance the prediction performance of the target field.
Introduction
While the limitations of simulation models make them unreliable for developing unconventional reservoirs, the increasing number of wells drilled in recent decades has provided an extensive database for tight oil horizontal wells. The use of such large data sets has permitted the use of data-driven proxy models.
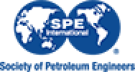