The advent of big data represents a major development in the industry, but the staggering amounts of data generated by recent methods is often not efficiently and effectively used. The increasing complexity of oil and gas systems as the result of nonlinearity, high levels of uncertainty, and the large volumes of data generated calls for more-sophisticated models that can turn raw data into actionable knowledge and are able to represent the complex relationships among the system-state (input, internal, and output) variables.
Data-driven methods and computational intelligence are being used increasingly as a complement or replacement for physics-based models such as numerical reservoir modeling and simulation. Although data-driven methods are effective in solving critical problems in the industry, design challenges exist that could create extra problems and could even render the model useless. Some focal points need to be described clearly, such as the optimum number of layers to solve a problem, the number of units needed for each layer, the generalization ability of the algorithm, and the boundaries of a training set to handle the problem successfully.
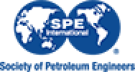