Almost every day, petroleum engineers are coming to realize that they’ve got an arsenal of good ideas on how to leverage large, messy data sets to add value to their businesses. Those who have enlisted in the Analytics Army have progressed from siloed digitalization attempts to well-concerted digital transformation strategies that reflect high levels of organizational digital maturity.
Paper SPE 220686hits an undeniable sweet spot for production and reservoir engineers, using an array of machine-learning methods to accurately predict real-time well status. Practicality here is evident, because only widely available surface-measured pressures, temperatures, and choke-valve positions are used to classify online and offline well status. The 99% accuracy levels achieved represent a boon not only for those interested in more-reliable rate allocation but also for well-integrity and flow-assurance applications.
With ever-increasing data acquisition volumes, data-labeling and categorization problems increase in lockstep. After all, how well can your luggage be found without proper tagging? Anyone remember the 2022 summer of lost luggage? The value proposition of paper SPE 218865 is clear, using a combination of natural language processing for coiled tubing operations reports and pattern recognition of multimodal job data to automatically label the job types and technologies used. A myriad of other cases spring to mind for repurposing the solution to transform current onerous processes of metadata generation.
In the aftermath of the explosion in popularity of all things generative artificial intelligence, paper SPE 217671 steals the limelight. This paper exploits good data foundations by painting a roadmap to building your own drilling chatbot adviser. Compared with other generalized large language models, the zero-shot learning technique allows the chatbot to answer queries for drilling domain-specific knowledge that it hasn’t explicitly “seen.” The authors have even publicized the training data sets collated from the Norwegian Petroleum Directorate to enable quick and relatively inexpensive replication.
This Month’s Technical Papers
Real-Time Well-Status Prediction Techniques Use AI for Accurate Rate Allocation
Zero-Shot Learning With Large Language Models Enhances Drilling‑Information Retrieval
Automated Framework Labels CT Job Type and Technology for Large Operational Data Sets
Recommended Additional Reading
URTeC 4045912 Using Machine Learning To Automate Fracture‑Driven-Interaction Analysis by Reid Thompson, Momentum AI, et al.
SPE 220833 Preliminary Research on Applications of Large Language Models in the Exploration and Production Industry by X.G. Zhou, PetroChina, et al.
SPE 220714 Automated Well and Reservoir Management Using Hybrid Physics and Data-Driven Models—Case Study by Azreen Mustafa, Hess Corporation, et al.
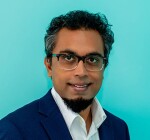
Kamlesh Ramcharitar, SPE, is a data and insights analyst with the Northern Alberta Institute of Technology (NAIT). He worked at Shell Trinidad and Tobago as a technical data manager before moving to the NAIT. Ramcharitar has more than 15 years of experience in reservoir, production, and process engineering across multiple private and state-owned companies. He holds BS and MS degrees in chemical and petroleum engineering, respectively, from the University of the West Indies. Ramcharitar has published SPE papers on synthesizing sonic log data sets and applied machine learning for inferring subsurface compartmentalization. He has been awarded the Regional Young Professional outstanding service award and served as technical program chair for the SPE Energy Resources Conference and on the Trinidad and Tobago section board.