In 1957, the Journal of Petroleum Technology published an article titled “Application of Large Computers to Reservoir Engineering Problems.” That was the first reference in JPT to what became known as supercomputers.
The high-speed computers used in the 1957 article were capable of performing 60 million operations in about 3½ hours. They were proposed to analyze the thorny problem of multiphase flow. “It presently appears that the large computer will be required to investigate multiphase flow and to predict the flow behavior of oil and gas reservoirs considering two- and three-space dimensions.”
Now, 67 years later, supercomputer speeds are measured in trillions of operations per second, and investigating multiphase flow is just one of the many uses. This extreme growth in speed has given rise to artificial intelligence (AI) and machine learning (ML), which has found its way into almost every corner of the petroleum industry.
The Society of Petroleum Engineers and JPT has kept up with the digital advances in the industry, holding countless conferences, symposia, and other meetings centered on the digital aspects of the industry and recently creating the Data Science and Engineering Analytics (DSEA) technical discipline. In 2019, SPE launched the Data Science and Digital Engineering in Upstream Oil and Gas online publication.
Four leaders in the oil and gas digital space shared their views on the current state of data science in the industry and the future of the discipline.
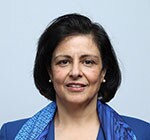
Sushma Bhan, currently on the board of directors for Ikon Science, is SPE’s Technical Director for DSEA. Before joining Ikon, she worked for Shell for 32 years, eventually rising to the role of chief data officer for subsurface and wells.
“My journey in the oil and gas industry began in 1988, when I joined Shell’s Production Computing Assisted Operations team as a programmer analyst,” Bhan said. “During my time there, I gained valuable exposure to field production operations and developed a deep understanding of real‑time data.”
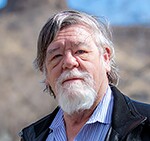
Jim Crompton, who is mostly retired, is an affiliate professor of petroleum engineering at the Colorado School of Mines, a faculty fellow at the school’s Payne Institute for Public Policy, and director of Reflections Data Consulting.
“My academic career started out in exploration geophysics, so my first introduction to engineering analytics came from processing seismic data at Chevron Geophysical Company in Houston,” Crompton said. “I studied earthquake seismology in graduate school, but, when I graduated, I learned the oil and gas industry paid a lot more than the USGS did, so my career goals changed.”
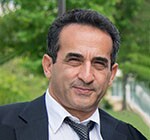
Shahab D. Mohaghegh is a professor at West Virginia University and the president of Intelligent Solutions.
“Since I started working for the petroleum industry throughout the world using artificial intelligence in 2000, I was able to work with actual data (field measurements) that has been saved by all the companies,” Mohaghegh said. “Working with actual data using AI provided several technologies that have been incredibly fantastic compared to what we have done in the past.”
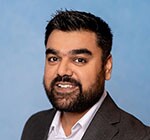
Pushpesh Sharma, the chair of the DSEA Technical Section, holds a PhD degree in chemical engineering from the University of Houston and is senior product manager for Aspen Technology.
“Till a few years ago, the focus was on proving the efficacy of data science/machine learning methods for energy use cases,” Sharma said. “However, in recent years, the concerns are around large-scale deployment, maintenance, and the black-box nature of ML models. Because of that, I started seeing increased focus on the deployment, explainability, and trust of ML models.”
JPT: How have advancements in technology affected the discipline? Are there any technologies that you find particularly promising for the future?
Bhan: In recent years, technological innovations have significantly impacted the oil and gas sector. These advancements span system architecture, computing power, and software development, and they have broadened the applicability of data science across all aspects of the industry.
- Mainframes (IT Systems) to Mobile Devices—Early in my career, SCADA [supervisory control and data acquisition] systems marked a turning point. Since then, we’ve witnessed remarkable progress in data speed and reliability. Over the past 3 decades, we’ve transitioned from massive mainframe computers and supercomputers to compact mobile devices capable of managing field operations. Mobile handheld devices now possess high computing power that was unfathomable just a few decades ago. Additionally, data visualization and graphics quality have improved significantly. The shift from desktops to laptops and mobile phones has revolutionized accessibility, especially in remote, high-risk, or challenging geographical locations.
- Cloud Computing and Data Integration—The advent of cloud computing has transformed data accessibility. Enormous volumes of data (e.g., seismic and real-time sensor data) from various systems are now being seamlessly integrated, enabling rapid decision-making.
- Artificial Intelligence (AI) Potential—AI has been around since the 1950s; however, recent developments like OpenAI’s Generative AI have sparked renewed enthusiasm. Yet, the quality and readiness of underlying data remain critical. The applications of AI are becoming common. I was impressed by a recent software application customized using thousands of documents for speedy information and knowhow extraction for exploration business (vs. text search)—also, AI’s usage for drilling accuracy and seismic interpretation. As our industry relies on historical and legacy data, ensuring robust data access and quality assurance is critical for success before expecting reliable results from algorithms and standardized models.
The convergence of innovative technology, data science, and AI hold great promise for driving efficiency, accelerating digital transformation, improved sustainability focus, and timely informed decision-making across the industry. As we move forward, let’s prioritize data readiness for AI, integrity, and its easy access to unlock the full potential of data sciences to support affordable worldwide energy needs.
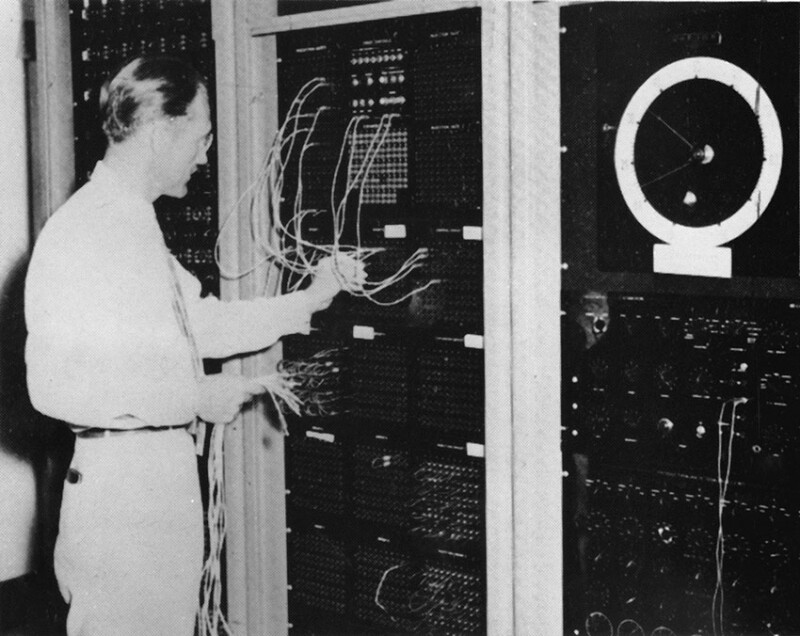
Crompton: The technology foundation of the digital oil field started with advances in sensors, followed by communications and networking in often challenging field (and downhole) conditions including internet-of-things devices and cloud computing. Advances in computing from data center scale like high-performance computing and GPU [graphics processing unit] chips to small-scale processing you can carry with you on your laptop allow more data and larger models to be developed.
Advances in data visualization and in the human/machine interface help the human engineers to interact better with all this data. But, of course, data quality and the lack of comprehensive data standards is a constant and persistent barrier. In the future, artificial intelligence/machine learning and generative AI will help us build larger data-driven predictive models, but the challenge is will they be accurate enough to make better decisions. That is a data quality challenge as well. Finding the best, trusted data to use in developing a model is the key step in machine learning, not which algorithm you use.
Engineering analysis is not new to the petroleum engineering discipline. Advances from the last century have led from computational fluid dynamics to mass balance to gas composition analysis to decline curve analysis to modern machine-learning-based automated drilling solutions. And there will be further advances in the days and year ahead.
Sharma: DSEA, being the Data Science and Engineering Analytics discipline, is always on the forefront of technology because the nature of the data science domain is agile. Almost every day, you hear about a new machine learning algorithm or a new type of data viz service. But I think the biggest change for the DSEA discipline is the advent of generative AI, more specifically large language models (LLMs; e.g., ChatGPT). LLMs have captured the imagination of the global population. A lot of major companies have started working on their version of ChatGPT, including domain specific LLMs. In addition to LLMs, generative AI opens up a variety of other use cases that were unthinkable before, such as generating data/images/content. Generative AI, in my opinion, is the most promising technology for the discipline.
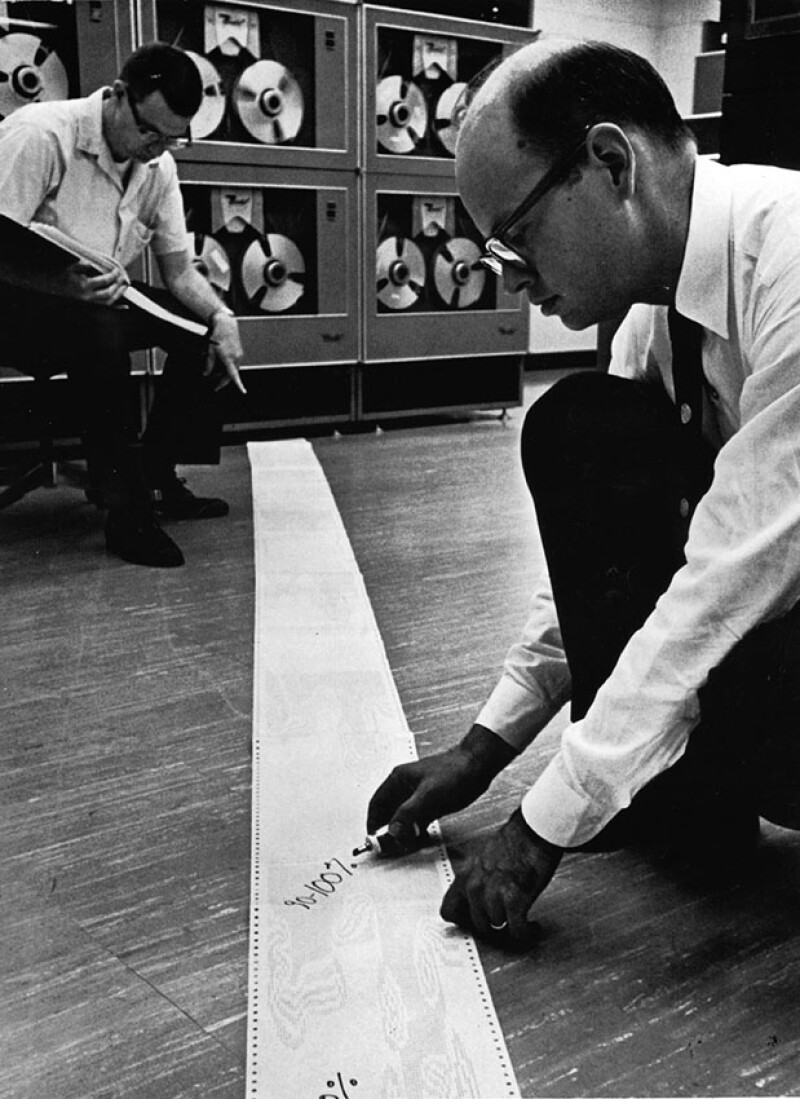
JPT: What do you think are the most pressing challenges and opportunities facing the discipline today?
Bhan: The Data Science and Engineering Analytics discipline faces dynamic challenges and opportunities that evolve with the business climate. In my view, here are the current challenges:
Hybrid Skilled Talent Availability—To meet the demands of this discipline, we need professionals with a blend of expertise in data sciences and petroleum engineering. These individuals must also grasp the latest advancements in computing technology and understand the other related upstream disciplines and the business needs—for example, the need to achieve successful production (exceed past metrics) from early discovery in half the time it was done in the past.
- Data Management—Managing vast volumes of critical data remains crucial for achieving reliable results. Business ownership, sustained support, skilled resources, and investments are essential components of effective multidisciplinary technical data management.
- Data Integration—Simplifying processes and ensuring seamless data flow are necessary for integrated systems and driving successful digital transformation or end-to-end realistic digital oil fields.
- Compliance—Adhering to legal and security requirements is non-negotiable at both organizational and individual levels. It’s key for the industry to be actively involved in shaping these industrywide international laws and regulations.
There are tremendous opportunities, particularly in automation of day-to-day manual work flows or field maneuvers. For instance, analyzing large data volumes with higher accuracy and precision can replace human errors, as seen in seismic interpretation; eliminate geohazards; lower carbon footprint; and enable predictive analytics used in field operations. DSEA’s progress will be propelled by navigating the challenges while leveraging opportunities to showcase business impact.
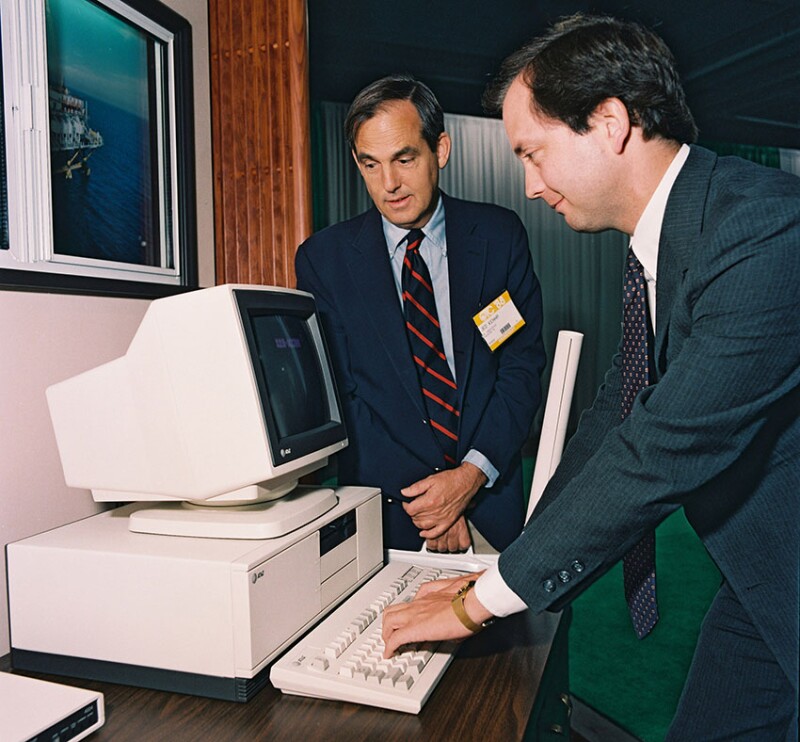
Crompton: I have always had a slide on this topic in my digital oilfield talks. It hasn’t changed much in over a decade. This is my list of challenges:
- Security—There have been many different data breaches, and combining different data sources can be difficult and complex to keep secure for the outside world. Too often, large companies have been hacked and personal data has been stolen. Getting this right is difficult.
- Budget—Planning the budget for a big data project is difficult because of the many unknowns—which technology to use, how to use it, what to implement on premises versus what to use from the cloud. All these questions affect your budget.
- Lack of Talent To Implement and Run—This is a major issue for any organization. big data talent is scarce and, therefore, expensive. Companies that want to move forward with big data should think of this carefully. There are several options available ranging from hiring consultants, working with software-as-a-service companies, or training your own staff.
- Integration With Existing Systems—Many organizations have legacy systems that need to be incorporated. In addition, many organizations have their data in silos across the organization. Getting this fixed and integrating new big data technology with existing systems is a challenge.
One new challenge I can see is that many new data sources come from statistical measurements, so the truth is no longer deterministic but probabilistic. We must remember our training in uncertainties (as opposed to measurement error) and probabilities to understand the data-driven models we are developing.
Mohaghegh: It seems that the challenges today have to do with marketing and business, not with science and technology.
Sharma: The biggest challenge for the DSEA discipline is the inability to capture the business value from ML use cases. We see a lot of AI applications in small scale, but they never make it to large-scale deployment. There are various reasons behind that, but, briefly, the top three are:
- Absence of Data Culture—Organizations need to set up a culture for AI and experimentation.
- Data Access and Pipelines—Availability and accessing the data is still the biggest problem with most AI applications.
- Explainability—ML models are considered black box in nature. It is hard to trust the results of a model if we can’t explain it. This also includes the absence of constraints in model outputs.
There are a lot of ideas out there to address these issues, but they still are not widely applicable.
JPT: Where do you see the discipline heading in the next decade? Are there any emerging trends or technologies that you think will shape the future?
Bhan: The DSEA discipline is poised for significant growth opportunities within the oil and gas industry. I anticipate the most substantial impact in the following areas:
- Process Multidisciplinary Integration—Breaking down system and data silos to enable end-to-end value generation. This includes shortening the discovery-to-reserves development cycle and implementing just-in‑time optimal well interventions for additional production.
- Automation With AI and Robotics—Streamlining manual processes through automation, leveraging artificial intelligence (AI) and robotics.
- Remote Operations and Management—Utilizing efficient visualization and control devices for remote operations, thereby minimizing staffing requirements and enhancing safety efforts.
- Enhanced Transparency on Emissions and Carbon Footprint—Facilitating easier data access, analytics, and adherence to industry standards and benchmarks related to emissions and carbon footprint.
These trends align with the broader technological advancements unfolding today, including generative AI, which has the potential to transform businesses across various industries. As we move forward, understanding these trends and systems interdependencies, mitigating potential risks, acquiring the necessary skilled global talent and legal-regulatory, including ethics compliance, will be crucial for organizations to harness their full potential.
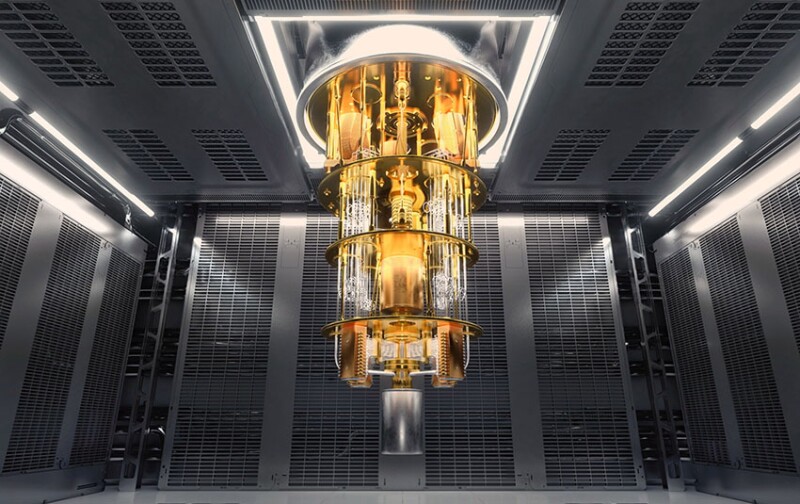
Crompton: The digital oil field is a reality:
- Field automation
- Real-time drilling and production systems
- Earth and reservoir modeling
- Collaboration and visualization
New engineers and Earth scientists are entering the workforce with high digital literacy and some training in programming. Petroleum engineering and Earth science “intellectual property” comes in the shape of software. “Innovation at the edge” comes from working on projects; the impact of central research groups is decreasing. New technology developments come from the outside, so innovation is more important than invention. Scaling pilot solutions to enterprise scale is a challenge. Significant gaps continue to surface (lack of reuse, fragile integration, poor data foundation, lack of end-to-end system design).
Mohaghegh: I am sure that AI will change everything in petroleum engineering and move it incredibly better than it has till now.
Sharma: The DSEA discipline will stay key to profitability due to its implication in energy transition, sustainability, and efficiency. As I mentioned before, generative AI will be an important player in the future. There are certain questions that we would need to answer as a discipline.
- How do we make data more accessible?
- How do we create benchmarks for AI applications?
- How do we trust the results of a machine learning model?
- How do we create guardrails around the misuse of AI?
- How do we foster human creativity in the age of generative AI?
For Further Reading
SPE 108206 Smart Fields—Optimizing Existing Fields by Frans G. van den Berg, Shell.
SPE 170986 Technical Data Management—Standards and Replication for Enabling Increased Production From Global Assetsby Sushma Bhan, Shell.
SPE 166339 Integrated Data Visualization With E-WellBooks: Gaining Efficiencies To Enable Production in the Gulf of Mexicoby Sushma Bhan, Raisa Kunichoff, Majeed Yousif, Lyndon Tiu, and Gary Leist, Shell.
SPE 96390 Real-Time Asset Management: From Vision to Engagement—An Operator’s Experience by T. Unneland and M. Hauser, Chevron.
SPE 167895 Intelligent Energy: The Past, the Present, and the Future, 2014 by Helen Gilman, Wipro, and Jan-Erik Nordtvedt, Epsis.
SPE 127715 The Future of Integrated Operations by Jim Crompton, Chevron, and Helen Gilman, SAIC.