Reservoir history-matching is essential for understanding subsurface uncertainty in rock properties. However, conventional history-matching methods usually require numerous forward model evaluations and rely heavily on the previous estimation of uncertain model parameters. To resolve these issues, the authors write that they resort to cutting-edge deep-learning (DL) technologies for their universal approximation capability in forward and inverse modeling based on automatic differentiation. In the complete paper, the authors develop a deep neural network-based history-matching (DNN-HM) workflow with enhanced efficiency and scalability for solving complex history-matching problems.
Methodology
The developed DNN-HM workflow consists of a forward modeling part for two-phase waterflooding reservoir simulations and the inverse modeling part for history-matching tasks.
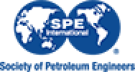