Everywhere you look these days, there is talk of how advances in big data, artificial intelligence (AI), and machine learning (ML) will revolutionize virtually every aspect of our lives. The thought of letting computer algorithms pore over mounds of data collected every day and spit out solutions to our biggest problems is certainly appealing. This month, we will look at how researchers in the drilling domain are using this potential to improve well construction.
I would be remiss if I did not caution the reader to maintain some healthy perspective when looking at how other areas have been changed and assuming that a direct analog exists for drilling. Consider this: It is estimated that there are fewer than 100,000 wells drilled each year, meaning that more data is generated by traffic in a small city in a day than is produced by the entire global drilling industry each year. Suffice it to say, while AI and ML are powerful tools that will certainly effect change, one should not expect off-the-shelf solutions to simply be plug-and-play for revolutionizing drilling. Instead, it will be careful application of these tools combined with a strong grounding in the fundamental physics and engineering of the domain that will lead to meaningful progress.
I urge readers to keep this in mind as they peruse the selection of papers for this month. Each takes a different approach to how problems are solved with data and algorithms, but they all share a common thread: There must be a solid foundation in domain knowledge to build a successful solution. Much like a sledgehammer, AI and ML are powerful tools, but they are not the right tool for every job. The key is knowing exactly when and how to use them in conjunction with everything else in the toolbox to achieve the maximum benefit.
This Month’s Technical Papers
Combined ML- and Physics-Based Techniques Achieve Real-Time Stick/Slip Mitigation
Methodology Prevents Drillstring Fatigue and Failure in Deep Well With Large Shallow Dogleg
Study Looks at Well Construction as Engineering With Data
Recommended Additional Reading
IPTC 24078 Real-Time Detection of Stuck Pipe Using Hybrid AI/Physical-Prediction Models by Mohammed A. Malki, Saudi Aramco, et al.
SPE 217953 Drillstring Failures: Inevitable or Not? by G. Plessis, NOV, et al.
SPE 220690 Automating Drilling Practices To Compensate for Interactions Between Tool Joints and the Rotating Head by David Forrest, Precision Drilling, et al.
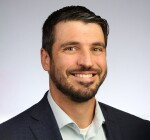
Marc Willerth, SPE, is a research and innovation manager at Helmerich & Payne, overseeing a research team working on wellbore positioning, drilling simulation, condition-based maintenance, and integrated drilling/geology solutions. He holds bachelor’s degrees in chemistry and chemical engineering from Purdue University. Willerth joined Helmerich & Payne in 2017 as part of the MagVAR acquisition and, before that, worked for Scientific Drilling and SLB. He has more than 20 publications and patents, primarily involving drilling, geology, and wellbore placement. Willerth is an active member of SPE and currently serves as chair of the Error Model subcommittee of the SPE Wellbore Positioning Technical Section and is on the JPT Editorial Review Board.