The complete paper presents an artificial intelligence (AI) algorithm the authors call dual heuristic dynamic programming (DHDP) that is used to solve optimization-control problems. Fast, self-learning control based on DHDP is illustrated for trajectory-tracking levels on a quadruple tank system (QTS) consisting of four tanks and two electrical pumps with two pressure-control valves. Two artificial neural networks are constructed for the DHDP approach: the critic network (the provider of a critique or evaluated signals) and the actor network or controller (the provider of control signals). The DHDP controller is learned without human intervention.
Approximate Dynamic Programming (ADP)
Recently, many different types of artificial algorithms have been applied in petroleum fields to solve optimization problems. This complete paper introduces a new field of AI applicable to oil and gas, ADP. ADP is a useful tool to overcome the behavior of nonlinear systems and is a special algorithm of reinforcement learning (RL). The authors write that ADP can be viewed as consisting of three categories: heuristic dynamic programming (HDP), DHDP, and globalized HDP. ADP features two neural networks—an actor and a critic—to provide an optimal control signal and long-cost value, respectively.
ADP has numerous applications.
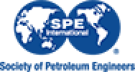