Failure to prioritize objectives and improper selection of candidate wells can have significant implications for both derived value and potential risk. This paper addresses the business problem of reducing the uncertainty of well-work-program outcomes so that more-informed choices can be made, enhancing the benefits and value of a well-work program. It illustrates the use of data-driven models to estimate key performance indicators for well-work jobs and to predict the likely outcome using predetermined success criteria.
Introduction
Well work consists of the complete end-to-end business process covering any operation on an oil or gas well during or at the end of its productive life. Well-by-well reviews, with good support information, remain the best way of spotting large amounts of potential production.
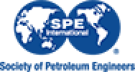