In the complete paper, the authors present exploratory data analyses to evaluate comprehensively the performance of a steady-state multiphase-flow point model in predicting high‑pressure, near-horizontal data from independent experiments. This effort provides wide-ranging insight that can reflect the current state of the art of multiphase-flow modeling and pinpoint areas where improvements are needed. Much of the complete paper is devoted to the literature relating to the authors’ work; these specific citations are not included in this synopsis.
Introduction
The emergence of “big data” has encouraged the use of data from various sources to enhance the decision-making process. The authors write that, unfortunately, multiphase-flow studies often are performed in theoretical silos, within which specific experiments were performed and upon which certain model improvements were proposed.
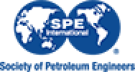