I recently read about an archeological study at Interamna Lirenas, central Italy, where a very ordinary Roman settlement; thought to be unpromising, ‘a failed backwater,’ revealed new discoveries that have forced historians to rethink what they believed to be true about the final years of the Roman Empire. The study revealed a town that was thriving for centuries after Roman Italy was thought to have been in decline.
The study’s author and project lead said, “There was nothing on the surface, no visible evidence of buildings, just bits of broken pottery. But what we discovered wasn’t a backwater, far from it. We found a thriving town adapting to every challenge thrown at it for 900 years.” The project lead goes on to say that many other average Roman towns in Italy were just as resilient, “It’s just that archaeologists have only recently begun to apply the right techniques and approaches to see this.”
The story reminded me of the enormous variety and volume of historic data that’s been inaccessible for decades in our industry, trapping knowledge and insights of enormous value. This data is old, but it’s not out of date. In truth, it’s a nice problem to have. If we apply new techniques, we can open a world of new information about an asset, operation, or enterprise.
The data includes commercial field development planning, well logs, drilling reports, consulting, unstructured data reports—all the things that combine with the structured technical information that we get from surveys and planning activities.
This treasure trove of data is untapped due to various reasons—outdated infrastructure, fragmented systems, storage in filing cabinets, siloed databases, and forgotten spreadsheets … bits of broken pottery, if you will.
Often, it’s used once and then never referenced again.
Organizations are wrestling with the challenge of bringing this old data into their business. They need to be able to look at the information, to recognize what it is, know what it means, where it should be filed away. They need to be able to bring it together with other relevant information and contextualize it.
The end goal is to have it available when people come to act, to make decisions. For this, it must be reliable, trusted, and discoverable—and at their fingertips. By the time it reaches people, or artificial intelligence (AI), it must be ready to use.
Connecting Data
One challenge companies face is the mechanical issue of connecting the data—getting it out of these siloed and fragmented systems—and into a system where it can be parsed and understood. Thankfully, in recent years a swathe of good technology products for achieving this at scale have been developed.
Data archaeology in our industry is now a realistic prospect.
The launch of Open Group’s OSDU Data Platform in 2021 was a giant leap forward. It standardizes how organizations manage data, which substantially lowers the costs of data management and increases the number of applications and solutions that can access and use the same data. Using a common standard enables companies to accelerate their adoption of new digital technology.
The platform provides the foundation, and because it is open, organizations have the freedom to augment the basic capabilities by building enhanced solutions. In recent years there have been huge advances in AI-enablement, generative AI, and cloud capabilities, and these can be built into enhanced OSDU-compliant platforms.
Leveraging AI
Generative AI has changed the game in unstructured data ingestion. We can use it to go back and look at data that was previously unreadable due to file formats or locations where converting it into usable data would require excessive time.
For example, take the process of analyzing drilling risks for upcoming wells. To do this you need to include insights from offset well reports, but operators commonly have different report templates, and the drilling events may be blocks of text in daily drilling reports (DDRs). For an engineer to find the hidden risks, they would need to read hundreds of DDRs. Generative AI can be easily trained to parse and extract this information in a fraction of the time and cost.
Advances in AI also help us extract more information from existing structured data. Let’s consider the economics for frontier exploration studies. These usually rely on images derived from 2D seismic acquisition configurations designed to sparsely cover a large area of exploration interest. The primary use of these images is to reduce the risk of a prospect before conducting 3D seismic studies, which are associated with much larger upfront financial commitments in acquisition and processing. As such, it’s highly valuable to reduce the gap between sparse 2D and 3D seismic studies.
Today, using machine learning, 2D seismic datasets and images can be converted into plausible 3D seismic volumes of the subsurface, thereby extracting insights from the area of interest. This cost-effective alternative approach improves the regional subsurface structural understanding of the prospect in large areas or in places where only 2D seismic images are available. This process has great potential in early hydrocarbon exploration, reservoir production management, geologically sequestered CO2 monitoring, and offshore windfarm high-resolution site characterization.
Bringing Context
The ability to find and contextualize related information from multiple different sources is another exciting benefit of using AI to reprocess old data. In the archeological dig, say we want to know about the lives of the people that lived in the Roman settlement. AI, in particular generative AI, can facilitate the connection between different sets of data that are related, thus creating the context and helping to tell the story. So you may have history books already written on that region. There will be geographical studies, analysis of various materials found in the dig such as cloth, ceramics, tools, and jewelry. All this data can be scattered away in different pieces of information, but with contextualization, it can be used to identify patterns that enable us to see back in time and gain a new perspective on the settlement and the people that lived there.
Generative AI can do this because it is trained on an incredibly broad set of different contexts, and this means it can recognize related information. It understands the semantics and can reason—link clues together—to find hidden links and create a fuller picture.
In our industry this could be related to a choke problem or a problem in a pipeline. With the help of the full context, you may be able to pinpoint the reason for a decline in production over a certain area and reverse it.
By running old data through the right applications, you can start to pull patterns and trends to derive new insights. The end goal isn’t a data platform that’s just concerned with data that resembles broken pieces of pots, it’s not just data archeology, or historic data. It’s a continually updating environment that represents the state of the enterprise, its operations, and its plans that can be constantly referred to for taking action and accelerating businesses forward.
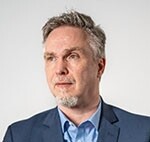
Jamie Cruise is director of the data business line at SLB. He has over 25 years’ experience in delivering transformative solutions for upstream data management for both corporate and government clients.