This year’s history matching and forecasting selections cover topics that include a deep-learning framework to forecast spatial/temporal dynamics of CO2 mineralization in reactive rocks, productivity decline analysis using coupled full-field and near‑wellbore poromechanics modeling, and a reservoir graph network model for multiwell forecasting in unconventional reservoirs.
In paper SPE 216998, the authors present a deep-learning methodology to create surrogate models capable of predicting the precipitation of minerals during CO2 injection into deep saline aquifers. The authors state that this approach allows for the rapid assessment of the individual contributions of each mineral in the CO2 mineralization process.
A coupling scheme of a full-field reservoir model and a near-wellbore poromechanics model, its application on productivity index (PI) decline analysis for a deepwater reservoir, and the evaluation of the drawdown effect on well productivity is the subject of paper SPE 212200. According to the authors, the coupling scheme captured geomechanical damages, predicted PI trends with different drawdowns, and enabled identification of safe drawdown limits for operational decisions.
The authors of paper URTeC 3855422 present a hybrid approach that combines physics with data‑driven approaches for forecasting the performance of unconventional wells under codevelopment. As per the authors, the reservoir graph network model, which describes the drainage volume of an unconventional well using a set of 1D gridblocks parameterized by pore volume and transmissibility, provides an efficient way to model, history-match, and forecast the performance of unconventional wells by reducing the system complexity while maintaining the fundamental physics.
This Month’s Technical Papers
Deep-Learning Framework Forecasts Dynamics of Carbon Dioxide Mineralization
Poromechanics Modeling Forecasts Production, Analyzes Productivity Decline
Hybrid Approach Proves Effective in Multiwell Forecasting in Unconventionals
Recommended Additional Reading
URTeC 3862949 A Robust Workflow To Characterize Uncertainties of a Horizontal Well Pad: Multiwell History Matching for Unconventional Reservoirs by Chuxi Liu, The University of Texas at Austin, et al.
SPE 212668 Application of Simulation Opportunity Index and Assisted History Matching for Fishbone Completion Strategy in the Existing Single Horizontal Well in a Tight Gas Carbonate Reservoirby Bondan Bernadi, ADNOC, et al.
SPE 212723 Automated Production Forecasting Using a Novel Machine-Learning-Based Approach by Kaustubh Shrivastava, SLB, et al.
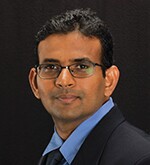
Gopi Nalla, SPE, is a senior reservoir engineer with DeGolyer and MacNaughton. He has 21 years of industry experience and has previously worked for 12 years with Chevron and 2 years with the Idaho National Laboratory. Nalla holds an MS degree in petroleum engineering from The University of Texas at Austin and a BS degree in chemical engineering from the National Institute of Technology Trichy, India. A licensed professional engineer in Texas and California, he serves on the JPT Editorial Review Board and as a reviewer for SPE Reservoir Evaluation & Engineering. Nalla can be reached at gnalla@demac.com.