In the past decades, the success of unconventional hydrocarbon resource development can be attributed primarily to the improved understanding of fracture systems, including both hydraulically induced fractures and natural fracture networks. To tackle the fracture characterization problem, several recent papers have provided novel insights into fracture modeling technique.
Because of the complex nature and heterogeneity of rock discontinuity, fabric, and texture, the fracture-modeling process typically suffers from limited data availability. Research shows that modeling results reached without interrogation of high-resolution petrophysical and geomechanical data can mislead because the fluid flow is actually controlled by fine-scale rock properties. A more-reliable fracture geometry can be obtained from an enhanced modeling process that preserves the signature from high-frequency data.
Advanced techniques to model fracturing processes with proppant transportation and thermodynamics require even more-sophisticated simulation and computation power. When the subsurface is too puzzling to be described by a physical model and existing data, machine learning and artificial intelligence can be adapted as a practical alternative to interpret complex fracture systems. Taking a discrete fracture network (DFN) as an example, a data-driven approach has been introduced to learn from outcrop, borehole imaging, core computed tomography scan, and seismic data to recognize stratigraphic bedding, faults, subseismic fractures, and hydraulic fractures. Input data can be collected by hand, 3D stereophotogrammetry, or drone.
When upscaling DFN into a coarse grid for reservoir simulation, deep-learning techniques such as convolutional neuron networks can be used to populate fracture properties into a dual-porosity/dual-permeability model approved to yield high accuracy compared with a fine-grid model. Furthermore, the new techniques greatly extend the application of fracture modeling in the arena of the energy transition, such as in geothermal optimization.
This Month’s Technical Papers
Machine-Learning Upscales Realistic Discrete Fracture Simulations
Integrated Work Flow Delivers Precise Properties Input for Unconventional Simulation
New Technology Yields High-Resolution 3D Modeling for Fracture Analyses
Recommended Additional Reading
SPE 203927 Numerical Simulation of Proppant Transport in Hydraulically Fractured Reservoirs by Seyhan Emre Gorucu, Computer Modelling Group, et al.
SPE 202679 Deep-Learning Approach To Predict Rheological Behavior of Supercritical CO2 Foam Fracturing Fluid Under Different Operating Conditions by Shehzad Ahmed, Khalifa University of Science and Technology, et al.
SPE 203983 A 3D Coupled Thermal/Hydraulic/Mechanical Model Using EDFM and XFEM for Hydraulic-Fracture-Dominated Geothermal Reservoirs by Xiangyu Yu, Colorado School of Mines, et al.
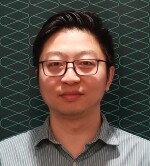
Junjie Yang, SPE, is a lead reservoir engineer with Baker Hughes. He has 8 years of experience in the oil and gas industry. Yang’s specialties include analytical and numerical reservoir modeling, hydraulic-fracture modeling, and optimization. He is also skilled in data analytics as well as integrated statistical analysis in production forecasting, rejuvenation candidate selection, and field-development strategy optimization. Yang has been leading and contributed to multidisciplinary consulting projects from major conventional and unconventional plays across North America, including superhot rock geothermal asset stimulation and development. He holds a PhD degree in petroleum and natural gas engineering from The Pennsylvania State University and is a member of the JPT Editorial Review Committee.