Upscaling of discrete fracture networks to continuum models such as the dual-porosity/dual-permeability (DP/DP) model is an industry-standard approach in modeling fractured reservoirs. In the complete paper, the author parametrizes the fine-scale fracture geometries and assesses the accuracy of several convolutional neural networks (CNNs) to learn the mapping between this parametrization and DP/DP model closures. The accuracy of the DP/DP results with the predicted model closures was assessed by a comparison with the corresponding fine-scale discrete fracture matrix (DFM) simulation of a two-phase flow in a realistic fracture geometry. The DP/DP results matched the DFM reference solution well.
×
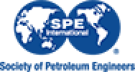
Continue Reading with SPE Membership
SPE Members: Please sign in at the top of the page for access to this member-exclusive content. If you are not a member and you find JPT content valuable, we encourage you to become a part of the SPE member community to gain full access.