Compositional reservoir simulation is a time-intensive activity demanding complex physics. In the complete paper, the authors review the advantages of machine learning (ML) in complex compositional reservoir simulations to determine fluid properties such as critical temperature and saturation pressure. An ML approach to predict critical temperatures during simulation based on the Heidemann-Khalil method is implemented, resulting in more-accurate results with lower computational cost, outperforming the standard method and improving performance on a giant field model with compositional gradient and miscible gas injection.
Field Description
The case study refers to a giant offshore carbonate field composed of multiple reservoirs. Production is currently in a rampup phase; crestal miscible hydrocarbon gas injection was implemented soon after startup.
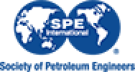