Routine well injection and production data contain significant information that can be used for closed-loop reservoir management and rapid field decision-making. Traditional physics-based numerical reservoir simulation can be prohibitive computationally for short-term decision cycles. As an alternative, reduced physics models often have a limited range of applicability. Pure machine-learning (ML) models often lack physical interpretability and can have limited predictive power.
×
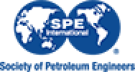
Continue Reading with SPE Membership
SPE Members: Please sign in at the top of the page for access to this member-exclusive content. If you are not a member and you find JPT content valuable, we encourage you to become a part of the SPE member community to gain full access.