The physics of fluid flow and transport processes in hydraulically fractured unconventional reservoirs is not well understood. As a result, predicted production behavior using conventional simulation often does not agree with observed field performance data. The discrepancy is caused by potential errors in the simulation model and the physical processes that take place in complex fractured rocks subjected to hydraulic fracturing. In the complete paper, the authors discuss the development of a deep-learning model to investigate the errors in simulation-based performance prediction in unconventional reservoirs.
Introduction
One of the major challenges for petroleum engineers working with unconventional reservoirs is a lack of models that accurately represent a physical relationship between the formation, completion and fluid properties, and production responses.
Statistical predictive models typically are used to extract sets of input parameters that better represent the properties of the field.
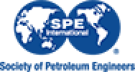