Permeability prediction or calculation in heterogeneous carbonate formations is a challenging task because of the complexity of rock properties and pore systems that are difficult to characterize accurately. In the study detailed in the complete paper, an efficient approach was developed and used to overcome this challenge by combining rock typing and machine-learning neural-network (MLNN) techniques to predict the permeability of heterogeneous carbonate formations accurately. The approach is applicable to a wide range of carbonate formations and has the potential to improve reservoir characterization and production optimization significantly.
Introduction
The reservoir under investigation offshore Abu Dhabi represents a complex amalgamation of sedimentary environments, ranging from tidal flat to lagoon and inner ramp, occasionally transitioning to midramp deposits. This intricate hierarchy of sedimentary cyclicity within the reservoir reflects the influence of diverse depositional processes and water-depth fluctuations, ultimately shaping the reservoir’s heterogeneity and dynamic fluid-flow pathways.
A notable geological feature within this setting is the prevalence of dolomitization, exerting a pronounced effect, primarily in the middle and lower sections of the reservoir.
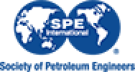