The sheer size of the Johan Sverdrup field development (expected recoverable reserves of 2.7 billion bbl, with projected operations of more than 50 years) has earned it designation as the operator’s digital flagship. The field has driven digital solutions and work flows that have the potential to be scaled up for the benefit of the operator’s entire portfolio. The complete paper describes the development of the “digital field worker” at Johan Sverdrup, an initiative that has changed the approach toward not only construction and completion but also operations.
Introduction
The field is approximately 150 km west of Stavanger in the North Sea in a water depth of 110–120 m (Fig. 1). The top reservoir is approximately 1800 m below mean sea level.
×
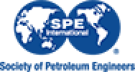
Continue Reading with SPE Membership
SPE Members: Please sign in at the top of the page for access to this member-exclusive content. If you are not a member and you find JPT content valuable, we encourage you to become a part of the SPE member community to gain full access.