Despite a recent industry emphasis on its effective use, data remains a largely untapped asset by operators around the world. One application with significant potential is the remote condition monitoring (CM) of production machinery and the use of CM to support a more proactive, even predictive, condition-based-maintenance (CBM) model. The complete paper discusses a remote-CM-enabled CBM model that uses artificial intelligence (AI) and machine learning (ML) on a North Sea platform operating in the Ivar Aasen field offshore Norway.
The proposed approach involves the creation of high-quality data by time-stamping data events. This data-sampling technique is driven by anomalies in equipment-performance-monitoring data. These data are sourced through the CM system’s linkages, with electrical, instrumentation, control, and telecom (EICT) built into the platform’s topsides structure.
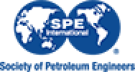