Accurately estimating gel strength is paramount for optimizing drilling operations and preventing cuttings from settling at the wellbore’s bottom. Traditional methods rely on rotational viscometers, which are time-intensive, equipment-dependent, and lack real-time monitoring capabilities. This study underscores the feasibility of leveraging machine learning (ML) as a practical tool for predicting drilling-fluid gel strength, offering real-time monitoring and precise predictions to enhance drilling efficiency, safety, and automation initiatives.
Background
The gel strength of drilling fluid is assessed using a viscometer, focusing on the 3-rev/min reading obtained after agitating the fluid at 600 rev/min to disrupt any gel formation. Initially, the measurement is taken when the mud reaches a static state for 10 seconds.
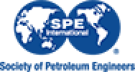