Among the sources of subsurface data, rock and fluid analyses stand out as the best means of directly measuring subsurface properties. The implication of modeling this data into an organized data store means better assessment of economic viability and producibility in frontier basins and the capability to identify bypassed pay in old wells that may not have rock material. The complete paper presents agile technologies that integrate data management, data-quality assessment, and predictive machine learning (ML) to maximize company asset value with legacy core data.
Introduction
The complete paper integrates data gathering, data filtering, the connecting of scattered data, and the building of useful knowledge models using legacy core data from various operating assets. This integration is achieved by a data quality check (QC) work flow and ML to improve the definition of reservoir rock properties that affect field development and asset management.
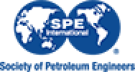