Petrophysical characterization in reservoirs with high heterogeneity is a consistent challenge. The case study presented in the complete paper describes a machine-learning (ML) technique to determine electrical properties. The methodology combines logs, rock types, and facies and digital core analyses from the Mamey field in northern Colombia, a reservoir composed of interlaminated mudstones and very-fine to fine sandstones enclosed in a deltaic environment and capped by cross-stratification sandstones associated with incised valley deposits. The results obtained indicate that the technique is feasible for estimating a continuous curve of the Archie parameters m and n associated with the textural changes identified in images and computed tomography.
Introduction
For many years, the industry has reviewed easily extracted reservoirs and neglected those that were slightly more complex.
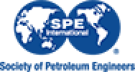