In this study, the authors investigated a fully data-driven approach using artificial neural networks (ANNs) for real-time virtual flowmetering and back-allocation in production wells. The main goal was to develop computationally efficient data-driven models to determine multiphase production rates of individual phases (gas and liquid) in wells using existing measured data in fields. The results showed that ANNs were capable of estimating multiphase flow rates accurately in both simulated and field data.
Introduction
Virtual-flowmetering (VFM) methods are categorized in two types generally: physics-based models (hydrodynamical approach) and data-driven models. In the physics-based approach, multiphase flow rates are estimated by simplified hydrodynamical models using sensor data (e.g., pressure and temperature) as input parameters.
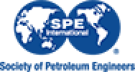