Drilling rate of penetration (ROP) is influenced by many factors, both controllable and uncontrollable, difficult to distinguish with the naked eye. Thus, machine-learning (ML) models such as neural networks have gained momentum in the drilling industry. Previous models were field-based or tool-based, which affected accuracy outside of the trained field. The authors of the complete paper aim to develop one generally applicable global ROP model, reducing the effort needed to redevelop models for every application.
Introduction
The authors have identified a need for an ROP model that can recommend parameters in real time, which ideally requires a general ROP model that can be applied with low prediction errors.
Formation properties are available in real time from logging tools; however, incorporating logging data into a global ROP model is challenging.
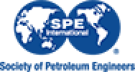