Saline aquifers and depleted hydrocarbon reservoirs with good seals located in tectonically stable zones make an excellent storage formation option for geological carbon sequestration. Ensuring that carbon dioxide (CO2) does not leak from these reservoirs is the key to any successful carbon capture and storage (CCS) project. In the complete paper, the authors demonstrate automated leakage detection in CCS projects using pressure data obtained from the Cranfield reservoir in Mississippi in the US. Results indicate that even simple deep-learning architectures such as multilayer feed-forward neural networks (MFNNs) can identify a leak using pressure data.
Introduction
Several methods that use different types of data currently are available to detect leaks. Although some of the methods are a direct indicator of CO2 presence, they cannot provide an early warning for the leaks, thus delaying remedial measures.
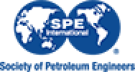