Nanoimaging techniques for characterizing pore-scale structure of shales trade off between high-resolution/high-contrast sample-destructive imaging modalities and low-contrast/low-resolution sample-preserving modalities. Acquisition of nanoscale images also is often time-consuming and expensive. In the complete paper, the author introduces methods for overcoming these challenges in image-based characterization of the fabric of shale source rocks using deep-learning models.
Introduction
A promising application of data-driven scale-translation techniques is nanoscale imaging. This application is important for studying shales because of the importance of nanoporosity in shale gas storage.
×
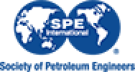
Continue Reading with SPE Membership
SPE Members: Please sign in at the top of the page for access to this member-exclusive content. If you are not a member and you find JPT content valuable, we encourage you to become a part of the SPE member community to gain full access.