New water treatment facilities in the Gulf of Mexico include a seawater sulfate removal unit (SRU) to mitigate reservoir souring and scaling. Current industrial practice relies on only pressure drop and regular cleaning intervals to perform SRU maintenance, which may result in reduced membrane life because of cleaning frequency or severe membrane fouling without the capability to predict fouling based on process conditions. The machine-learning techniques applied in the complete paper aim to deliver a prediction model based on both simulation and real-time field data. The model tracks and monitors system key performance indicators (KPIs).
KPI Model Establishment
Before KPI models were established in this study, key model input and output parameters were identified.
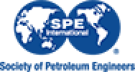