Because of their heterogeneity, carbonate reservoirs are more difficult to model than clastic reservoirs. The main difficulty comes from the number of different pore types, compared with the typical interparticle pore type in clastics. By using saturation-height models (SHMs) in combination with conventional permeability measurements, a new approach attempts to extract the fundamental properties of individual pore systems. The key idea centers on identifying the governing pore systems from capillary pressure curves and permeability measurements.
×
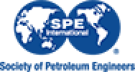
Continue Reading with SPE Membership
SPE Members: Please sign in at the top of the page for access to this member-exclusive content. If you are not a member and you find JPT content valuable, we encourage you to become a part of the SPE member community to gain full access.