For all that logging-while-drilling has provided since its widespread adoption in the 1980s, there is one thing on the industry’s wish list that it could never offer: an accurate way to tell the difference between oil and gas.
A new technology created by petrotechnicals at Equinor, however, has made this possible.
The innovation could be thought of as a pseudo-log, but Equinor is describing it as a reservoir-fluid-identification system. Using an internally developed machine-learning model, it compares a database of more than 4,000 reservoir samples against the real-time analysis of the mud gas that flows up a well as it is drilled.
Crunched out of the technology’s various hardware and software components is a prediction on the gas/oil ratio (GOR) that the rock being drilled through will have once it is producing. Since this happens in real time, it boils down to an alert system for when drillers are tapping into uneconomic pay zones.
“This is something people have tried to do for 30 years—using partial information to predict entire oil and gas properties,” said Tao Yang. He added that “the data acquisition is rather cheap compared with all the downhole tools, and it doesn’t cost you rig time,” highlighting that the mud-gas analyzer critical to the process sits on a rig or platform without interfering with drilling operations.
Yang is a reservoir technology specialist at Equinor and one of the authors of a technical paper (SPE 201323) about the new digital technology that was presented at the SPE Annual Technical Conference and Exhibition in October.
He and his colleagues spent more than 3 years building the system which began in the Norwegian oil company’s Houston office as a project to improve pressure/volume/temperature (PVT) analysis in tight-oil wells in North America. It has since found a home in the company’s much larger offshore business unit in Stavanger.
Offshore projects designed around certain oil-production targets can face harsh realities when they end up producing more associated gas than expected. It is the difference between drilling an underperforming well full of headaches and one that will pay out hundreds of millions of dollars over its lifetime.
By introducing real-time fluid identification, Equinor is trying to enforce a new control on that risk by giving drillers the information they need to pull the bit back and start drilling a sidetrack deeper into the formation where the odds are better of finding higher proportions of oil or condensates.
At the conference, Yang shared details about some of the first field implementations, saying that in most cases the GOR predictions made by the fluid-identification system were confirmed by traditional PVT analysis from the trial wells.
Unlike other advancements made on this front, he also said the new approach is the first of its kind to combine such a large database of PVT data with a machine-learning model “that is common to any well.” That means “we do not need to know where this well is located” to make a GOR prediction, said Yang.
After the conference, Equinor greenlighted the machine-learning tool for use in its Norwegian continental shelf program, where it is expected to help drill dozens of wells over the next 3 years.
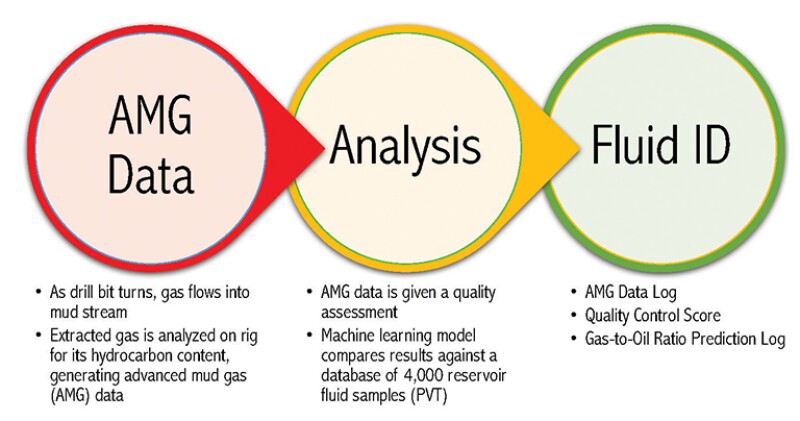
Filling in the Gas Gaps
A key aspect of the breakthrough involves the analysis of what is specifically referred to as advanced mud gas (AMG). As the drill bit moves through a fresh reservoir, gas breaks free and flows up to the rig along with the drilling-mud returns.
This mud gas has been used as a drilling tool of sorts for generations, but mostly just as an early-warning sign of an impending gas kick. In the digital age, companies have brought analyzers to the rig, where captured samples of mud gas are used to discern reservoir quality. This is the “advanced” component giving rise to the term AMG logging.
Despite the large number of AMG studies carried out over the years, industry literature reflects that past pursuits have been stymied by high error rates in the correlations.
“We’ve really changed that picture,” said Yang, who in 2019 was recognized with the SPE Distinguished Membership award, the organization’s top honor, for his work in reservoir-fluid characterization and improved oil recovery.
The problem that others have faced and that Equinor said it has overcome is that when mud gas finds its way to an analyzer on the rig, it is not all there. Yang likens it to having only a partial DNA sequence and then having to figure out which animal species it came from.
In this case, critical markers such as methane, butane, and pentane represent the building blocks of the DNA strain—and some are always missing. Equinor’s model pores over the PVT database with thousands of wells from all over the world (half of which comes from Equinor’s own data store with the other half from the geochemical specialist GeoMark Research) to put the missing pieces back in their place.
So, in addition to being able to tell oil apart from gas and quantify the ratio, the fluid-identification system can also tell what kind of gas is inside the wellbore. This is especially relevant in the offshore arena where even in a greenfield scenario, an operator often must run gas-injection schemes to sustain high reservoir pressures and promote a higher recovery factor.
If injection gas is present in the mud gas, the composition of which should be well-known to the operator, it strongly suggests that the gas is channeling too quickly through the formation and invading the new well’s production zone.
If an operator were to complete the well in this scenario, it will soon realize large sums will be spent on recycling produced gas from one well to another.
Breaking the Limits of Traditional Logging
The most accurate way to understand fluid content is by taking actual samples out of the reservoir. However, aside from high costs, the low sample volumes usually recovered during fluid sampling offer a limited resolution of the reservoir’s true makeup.
The next best thing is considered to be the neutron-density log—one of the most widely used downhole tools in the industry. For decades, operators have used the log to determine if what they are drilling into really is the oil reservoir.
But depending on reservoir conditions, the neutron-density log can be a misleading ally in the hunt for oil-rich rocks. The problem can be explained by simply looking at the log’s output.
When the two lines on a neutron-density log cross, it indicates a gas show. When they stay apart, it indicates the presence of oil-bearing rock. What the log cannot confirm, though, is whether what is inside that rock is residual oil (i.e., it is stuck to the rocks and not mobile) or oil that will easily flow into a wellbore.
In simple situations, a sandstone greenfield for instance, following the plots of the traditional log has led to success for many oil companies around the world. It is in the geologically complex reservoirs and those depleted by older wells that trusting the neutron-density log too much becomes a big risk—such as those aging assets in the North Sea.
The risk is compounded in horizontal wells where the drill bit must wind its way through different rock layers before hitting total depth. Furthermore, taking fluid samples from inside the lateral section of the well is not feasible with today’s technology, which leaves behind a major blind spot in the well’s most important area.
“If you’re dealing with different lithologies, then oil and gas cannot be simply differentiated by neutron-density responses,” explained Yang, adding, “If you don’t know, then you may complete the entire well and when you start to produce, there is a very high GOR.”
Lower GOR, Smaller Carbon Footprint
Aside from suboptimal economics, high-GOR wells can have other unwanted impacts, such as driving up topside costs and their carbon footprint.
The single largest source of emissions on a platform is the gas compressor used to send a portion of the product back into the subsurface for pressure maintenance. Drilling wells that produce higher ratios of oil means less gas-compression capacity is used, translating to lower CO2 emissions.
Yang noted that while the reservoir-fluid log was initially viewed as an exploration tool, these types of applications underscore that its biggest impact is likely to be on the development wells that come after the appraisal wells.
When development begins in an offshore field, the subsequent wells do not benefit from having nearly as much downhole data as the first. “And at the same time, your reservoir is becoming increasingly difficult to predict,” said Yang. “So, you have a small budget and very limited tools, and then your target is more and more complicated.”
After proving the technology, Equinor is keen on building up its library of AMG data. Prior to its development, Equinor had AMG data on about 30 wells across its international portfolio. Since the real-time identification project began, that figure has more than doubled.
What is most likely to slow down the rollout of the technology involves data quality. Equinor found its AMG data quality depended largely on three factors: the vendor, drilling events, and the efficiency of the mud-gas-interpretation process used to correct certain chemical ratios and generate a proxy for a true PVT sample.
As a result, only 70% of the AMG dataset it collected during field trials could be used for GOR predictions. Yang said it is hoped that improvements can be made in the near future to solve for these issues and make the system more reliable on future deployments.
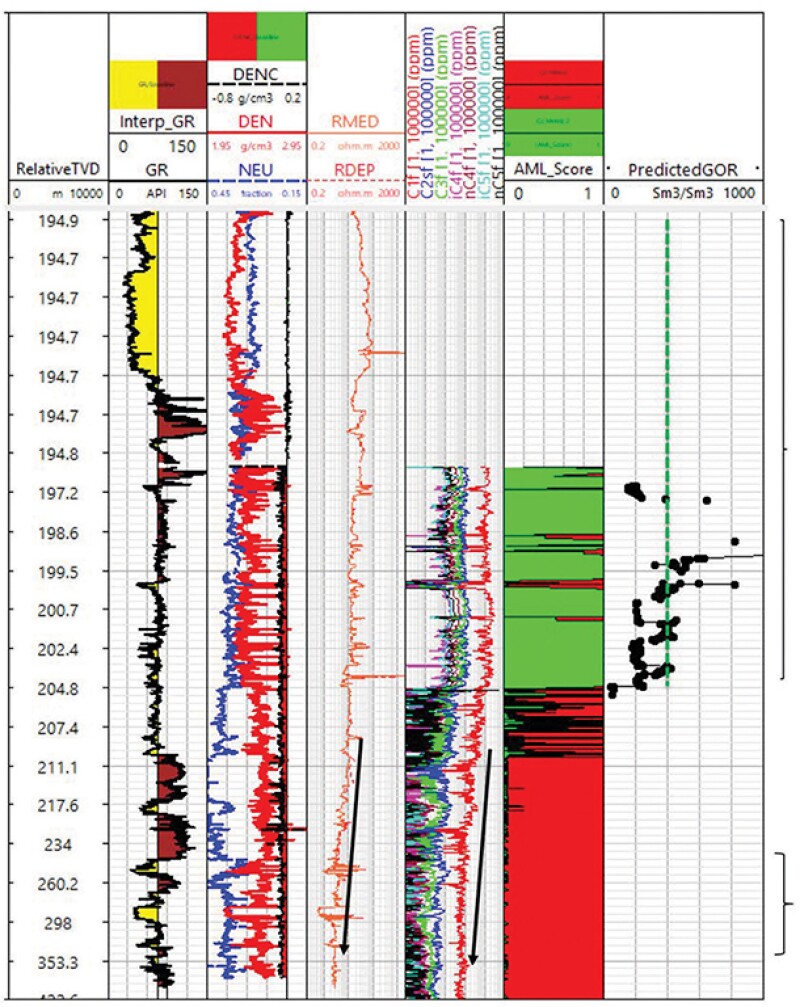
For Further Reading
SPE 201323 Real-Time Reservoir-Fluid Log from Advanced Mud-Gas Data by Ibnu Hafidz Arief and Tao Yang, Equinor.
SPE 195459 A Machine-Learning Approach To Predict Gas/Oil Ratio Based on Advanced Mud-Gas Data by Tao Yang, Ibnu Hafidz Arief, and Martin Niemann, Equinor et al.