Petrophysical work flows are designed primarily to process static data for traditional openhole logs, which can provide estimates of porosity, saturation, lithology, and mineralogy. However, these estimates normally have a high degree of uncertainty and formation testing and sampling (FTS) data often are required for reservoir-condition calibration. This paper bridges the gap between operational petrophysicists and FTS specialists, introducing an automated work flow by which petrophysicists can conduct FTS jobs.
Introduction
Wireline formation testers (WFTs) were commercialized in the late 1950s; drilling formation testers (DFTs) were introduced more recently. The primary benefits for FTS by means of wireline (e.g., WFT) or drillpipe (e.g., DFT) tools always has been a link between openhole log static volumetric formation evaluation and dynamic reservoir properties such as reservoir pressure, mobility, and fluid-sample composition, with many more applications under development using advanced testing tools and methods.
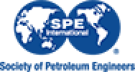