To place a well in the best possible reservoir zone, operators use geosteering to support real-time well-trajectory adjustments. Geosteering refers to the process of making directional well adjustments on the basis of real-time information acquired while drilling. This work presents a systematic geosteering work flow that automatically integrates a priori information and real-time measurements to update geomodels with uncertainties and uses the latest model predictions in a decision-support system (DSS). The DSS supports geosteering decisions by evaluating production potential against drilling and completion risks.
Introduction
This paper presents a consistent, systematic, and transparent work flow for geosteering. The starting point is a priori information, for example a probabilistic geomodel representing a geological interpretation based on surface seismic and logs from offset wells, including relevant interpretation uncertainties.
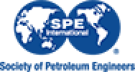