The evolution of computational technology, coupled with the ability to digitize hardware and engage with end users, has enabled machine learning to enhance operational efficiency, safety, and cost-effectiveness in offshore oil and gas development.
A key takeaway from recent literature is that, while automation tools have been present for years, their widespread adoption has been hindered by a lack of demonstrable financial benefits. To address this, the focus has shifted from mere deployment to achieving real value through significant cost savings, increased safety, and improved operational efficiencies (paper OTC 35137).
Automation efforts in onshore operations prioritize efficiency, while offshore automation must focus on risk reduction and minimizing nonproductive time. For instance, a cutting-edge vessel-motion-prediction tool integrates advanced machine-learning techniques with metocean forecasts to enhance the reliability and safety of offshore drilling operations (paper OTC 35341).
Paper OTC 35418 presents the importance of autonomous inflow-control devices in maximizing the production life of wells in deepwater West Africa, taking into account the various challenging reservoir conditions.
Another critical insight from the research is the role of human intervention in the deployment of automation systems. Successful deployment requires iterative adjustments and feedback from operators to tailor systems to the specific needs of offshore environments. Collaboration among engineers, operators, and decision-makers is essential in refining these technologies to align them with operational goals and safety standards.
Overall, these papers underscore the need for strategic adaptation of automation systems to the specific challenges of offshore environments. The success of predictive machine learning in this context depends on its ability to offer measurable financial benefits, improve safety, and drive operational efficiency.
This Month’s Technical Papers
Drilling Automation Serves as Engine of Change for Risk Prevention Offshore
Autonomous Inflow Control Devices Help Maximize Life of Wells in Deepwater West Africa
Application for Predicting Vessel Motion Enhances Operability in Offshore Drilling
Recommended Additional Reading
SPE 218473 Performance Optimization of Water-Injection Wells Using Autonomous Outflow Control Device Technology in Offshore Norway by G.F. Kvilaas, AkerBP, et al.
SPE 218360 Autonomous Restriction Navigation Through Wellbore in Wireline and Slickline Operations by S. DiPasquale, SLB, et al.
OTC 35394 Intelligent Optimization of Ultradeepwater Oil-Transmission Risers Using an Enhanced Graph Neural Network by Hong Zhu, China University of Petroleum-Beijing, et al.
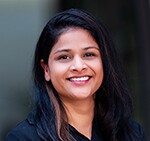
Swathika Jayakumar, SPE, is a technical manager with Core Laboratories. In this role, she leads a team of scientists and software program managers for the research and development of oilfield diagnostics chemicals and associated software. With 13 years of experience in the oil and gas industry, Jayakumar has partnered with clients worldwide to enhance hydrocarbon extraction efficiency using tracer diagnostics. She serves as an Executive Board member at the Society of Petroleum Engineers Gulf Coast Section and on the JPT Editorial Review Board. Jayakumar holds a master’s degree in petroleum engineering from Texas A&M University and an MBA degree from The University of Texas at Austin.