Automated artificial intelligence (AI) and machine learning (ML) workflows are helping ConocoPhillips quickly make better, faster expenditure decisions for its nonoperated Permian Basin assets.
While the Permian has prolific resources, factors such as well communication, spacing, and bench performance must be considered when planning how reserves are exploited, Tom Williford, a reservoir engineer at ConocoPhillips, said. As a partner in Permian Basin assets, ConocoPhillips needed to be able to rapidly screen opportunities and respond to the asset operator with an economically sound decision.
Williford told an Unconventional Resources Technology Conference (URTeC) audience on 17 June that using an AI- and ML-driven workflow enables ConocoPhillips to reach “adequate and technically robust analysis on each ballot decision” within the decision-making window so that as a partner, the company wasn’t making decisions “based on a deadline rather than economics” for assets that are operated by others (OBO).
Such assets are usually governed by joint operating agreements that require partners to respond in a certain window, usually 30 days, from the time an operator proposes well development activity, which may require considerable investment.
ConocoPhillips has a sizable OBO program and as such receives numerous drilling and workover proposals that must be answered in a timely fashion, Johan Daal, a Permian Basin staff reservoir engineer for ConocoPhillips, told the audience
And ConocoPhillips wants to reach the right decisions because profitability of Permian wells can be “off the charts,” he said.
Screening opportunities and returning investment decisions in 30 days or less involves “arduous work” considering the complex geology and reservoir conditions, multiple benches, stratigraphic variability, basin inversion, and the impact of fluid properties, Daal said. And the decisions are more complicated when factoring in multiple rigs, schedules, and expectations, he added.
ConocoPhillips needed to understand each development opportunity rapidly, assessing factors like how an operator is developing a formation, how many wells per section are being drilled, how wells will be flowed back, and how long they’ll take to reach peak production, Daal said, so ConocoPhillips developed an ML-based tool to help understand the performance of various scenarios.
The workflow, described in URTeC 4044067, calls on geological, completion, development, and performance data, and the “integration significantly elevates the decision-making process in unconventional reservoir development, spanning a wide array of tasks such as resource and reserve evaluation, field surveillance, development planning, and project decision-making.”
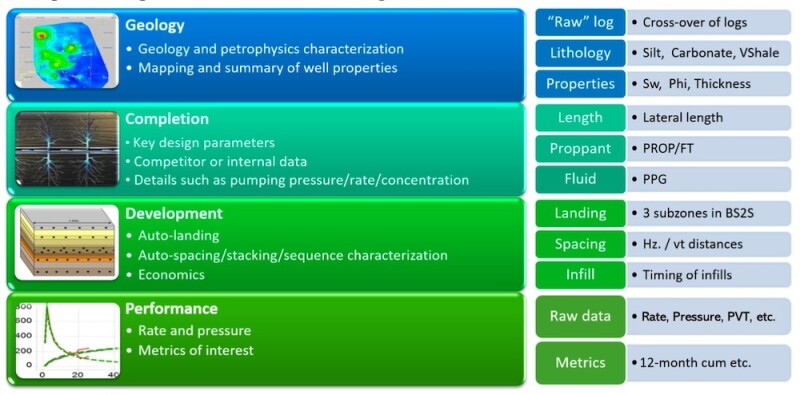
The workflow, informed by both engineering principles and physics, evaluates and constructs optimized development strategies designed to meet business objectives and constraints, according to the paper.
The automation reduces “decision turnaround from days to hours,” Williford said. “The workflow is not just ideal for nonoperated programs. It can also work for operated programs.”