Water affects almost every operation in the exploration and production industry. Until now, time-intensive laboratory tests or cumbersome third-party simulators were required to extract physicochemical properties. In the complete paper, a family of machine-learning-based reduced-order models (ROMs) trained on rigorous first-principle thermodynamic simulation results is presented. The developed ROMs that predict water properties enable automated decision-making and improve water-management work flows.
×
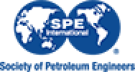
Continue Reading with SPE Membership
SPE Members: Please sign in at the top of the page for access to this member-exclusive content. If you are not a member and you find JPT content valuable, we encourage you to become a part of the SPE member community to gain full access.