The early days of artificial intelligence (AI) and machine learning (ML) were filled with promise, but now we’re witnessing a wave of practical applications transforming reservoir engineering. The integration of AI/ML with traditional workflows marks a turning point, unleashing the immense potential of these proven techniques to address our everyday challenges in reservoir simulation.
These advancements lead to more-accurate models and faster simulation turnaround times, allowing us to model various scenarios more efficiently. These techniques enable us to understand and simulate reservoir behavior with greater fidelity and focus on the underlying physics. This deeper understanding translates to more-confident decisions regarding reservoir management and development, with a clearer picture of potential risks.
Physics-informed machine learning emerges as a significant step forward, improving our understanding and providing better models while boosting runtimes, convergence, and overall performance. Paper IPTC 23730 highlights its application in tackling computationally intensive tasks such as critical temperature prediction with high accuracy, leading to significant speed-ups in simulations, particularly for complex compositional models undergoing miscible gas injection.
Paper IPTC 23935 presents a fundamentally different approach to reservoir simulation studies. Adaptive models use multiple smaller, specialized models instead of one giant model. These models can be developed and run independently, allowing for parallel workflows and significantly reduced turnaround times. Adaptive models foster a culture of rapid experimentation and iteration, aligning perfectly with the fail-fast approach—prioritizing the quick evaluation of ideas and discarding those that don’t show promise early on.
Paper SPE 214855 provides a comprehensive overview of a complex problem—wellbore modeling. This paper offers practical insights directly relevant to my work.
These advancements represent a significant leap forward, paving the way for more-powerful and -versatile reservoir models. As research and development continue, these techniques are poised to revolutionize how we manage and optimize oil and gas reservoirs. I hope you enjoy reading this selection of papers and find them enlightening.
This Month’s Technical Papers
Physics-Informed Machine Learning Applied to Complex Compositional Model in a Giant Field
Adaptive Approach for Reservoir Modeling Reduces Project Time, Improves Collaboration
Integrated Multiphase-Flow Modeling Technique Yields Accurate Downhole Pressure Predictions
Recommended Additional Reading
SPE 216722 Developing Consistent Relative Permeability and Capillary Pressure Models for Reservoir Simulation of Carbon Capture and Storage Projects by L.S. Lun, ExxonMobil, et al.
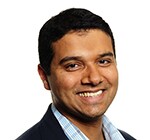
Anson Abraham, SPE, is a reservoir engineer at Canadian Natural Resources Ltd. (CNRL) with more than 17 years of experience in the industry. His professional journey has taken him through various reservoir engineering and simulation roles at CNRL, Computer Modelling Group, and SLB, as well as well testing and perforating while at SLB. Anson holds a bachelor’s degree in petroleum engineering from the University of Adelaide, a master’s degree in reservoir geosciences from the French Institute of Petroleum, and an MBA degree from the University of Calgary.