Using commercial numerical reservoir simulators to build a full-field reservoir model and simultaneously history matching multiple dynamic variables for a highly complex offshore mature field in Malaysia had proved challenging. In the complete paper, the authors demonstrate how artificial intelligence (AI) and machine learning can be used to build a purely data-driven reservoir simulation model that successfully history matches all dynamic variables for wells in this field and subsequently can be used for production forecasting. This synopsis concentrates on the process used, while the complete paper provides results of the fully automated history matching.
Subsurface Analytics
In the presented technique, which the authors call subsurface analytics, data-driven pattern-recognition technologies are used to embed the physics of the fluid flow through porous media and to create a model through discovering the best, most-appropriate relationships between all measured data in each reservoir. This is an alternative to starting with the construction of mathematical equations to model the physics of the fluid flow through porous media, followed by modification of geological models in order to achieve history match.
The key characteristics of subsurface analytics are that no interpretations, assumptions, or complex initial geological models (and thus no upscaling) exist.
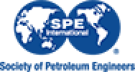