The Samarang field is located offshore Sabah, Malaysia. The field is undergoing a major redevelopment project with integrated operations (IO). In order to overcome a variety of challenges and to improve field awareness, several work flows were designed and deployed in order to achieve an early milestone of providing real-time well-performance monitoring, surveillance, and optimization. The paper discusses how these work flows were implemented in an integrated way to provide a modern decision-support system for the Samarang field.
Introduction
Samarang is an old oil field and thus exhibits the characteristics of many mature fields, including declining production. It has been in production for more than 35 years from multiple, now aging, platforms with insufficient metering and monitoring processes.
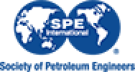