The project outlined in the complete paper demonstrates the feasibility of using deep-learning and machine-learning (ML) approaches to introduce camera-based solids monitoring to the drilling industry. Despite a short development time, the project proved that it was possible to recognize cuttings, cavings, and anomalies in the solids output using proprietary ML models and regular off-the-shelf hardware.
Computer-Vision Techniques for Cuttings Detection
Several technologies, such as 2D vision, stereo vision, structured light, or time-of-flight, can be used to detect objects such as drill cuttings on a shale shaker. Depending on the physics behind them, these techniques can recognize individual objects and their dimensions or generate depth maps by measuring the physical distance between the sensor and a defined point on the image.
Single 2D vision is the simplest and most cost‑effective approach but has limitations in measuring depth. Such cameras do not require any special sensors and can be directly installed close to the area of interest.
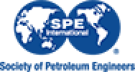