As the oil and gas industry forges a new landscape in the wake of the financial downturn, an expert argued that artificial intelligence (AI) technologies could potentially have a disruptive, valuable impact on operations in the near future.
In a webinar hosted by SeeAlgo, an AI consulting firm, Adnan Siddiqui discussed the ways in which AI and machine learning can improve project efficiency. Siddiqui, a principal at SeeAlgo, examined AI technology’s potential roles in several facets of oil and gas operations including project construction and fabrication quality assurance.
Siddiqui’s presentation coincided with a recent paper, “Enterprise AI Vision 2020 for Oil and Gas Capital Project,” co-authored with Tarlan Mammadov, an information management consultant and principal at DeepKnowledge. The paper outlined a plan to widely integrate AI into development projects by the year 2020.
Machine learning is an AI technique that allows computers to handle new situations through analysis, self-training observation and experience. The technique relies on the application of mathematical concepts, primarily statistics and linear algebra, to process, analyze, and predict future courses of action based on past data.
In recent years, various industries have built on the algorithms designed for machine learning to develop a subset of deep learning techniques that allow computers to make decisions about data in large multilayer neural networks. Some examples of deep learning systems include language translation websites, image search engines, and recommender websites like Netflix or Amazon, which use algorithms to discover hidden relationships in data and provide suggestions based on that data.
Siddiqui said a major challenge with any deep learning technique is that it requires significant amounts of data to be effective.
“The key is not really who has the better algorithm, but it’s who has the better data,” Siddiqui said. “That’s something for those of you who are evaluating and thinking about artificial intelligence. You need to be careful, as you look to explore other avenues, that you have enough data. If you don’t have enough data, the models will not really give you the promised performance.”
Siddiqui argued that machine learning can be utilized throughout the stage gate process for oil and gas projects, and that many organizations already have some of the AI technologies in place to integrate into the project development process.
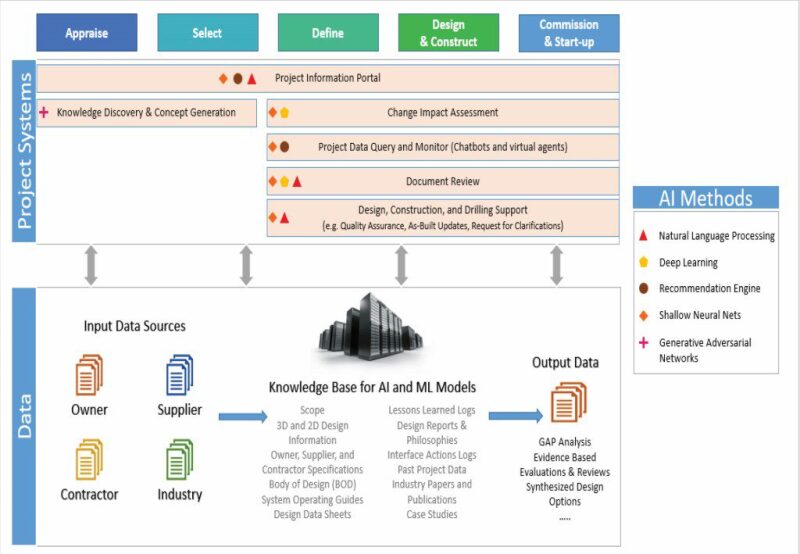
Fig. 1 illustrates stage gates for a typical project with the principal machine learning application opportunities listed at each step of the execution life cycle. Siddiqui divided the AI-enabled systems into two categories:
- Overall project productivity: project information management, knowledge discovery, and change impact assessment systems
- Discipline-specific tasks: management of design data consistency, document review, seismic interpretation, well drilling event prediction, and construction and fabrication quality assurance.
In their paper, Siddiqui and Mammadov argued that the use of machine learning for overall project productivity enhancement should help “make the sum of the individual parts that comprise the project more valuable.” To that end, AI systems would increase collaboration, knowledge sharing and discovery, and task integration. The use of machine learning for discipline-specific tasks would improve productivity, increase quality, and reduce the time required for tasks in specific disciplines such as facilities design.
Fig. 1 also outlines the data system for a typical oil and gas project using an AI model. Owners, suppliers, and contractors capture input data on documents and store them in servers, along with industry standards and regulations, and the output data include reports and evaluations like a gap analysis.
Siddiqui said many of the applications illustrated in Fig. 1 were traditionally done with a rule-based system or a system that did not require a lot of intelligence-gathering. However, as the figure shows, AI models would not force companies to employ a data system radically different from a traditional system.
“If we start looking at how we can use that [information] among the various stages of a project, you can start looking at classical systems, so you have project information portals. You look at discovering past knowledge and concept generation, change impacts, getting project data, reviewing the documents, and then support,” Siddiqui said.
Siddiqui and Mammadov listed several areas where machine learning models could have a transformative effect on the industry. For instance, with facility construction they estimate that these models can transform the content of 2D drawings and 3D models into a narrative format that subject matter experts (SMEs) can further analyze.
In addition, by quickly processing progress data and resource availability along with other information like health and safety observations, lessons learned, and construction deliverables, machine learning models can support SMEs in monitoring and auditing construction and fabrication quality.