Big-data analytics can allow a better understanding of a production system’s abnormal behavior. This knowledge is essential for the adoption of a proactive maintenance approach, leading to a shift toward condition-based maintenance (CBM). CBM focuses on performing interventions on the basis of actual and future states of a system determined by monitoring underlying deterioration processes. One of the building blocks of CBM design and implementation is the prognostic approach, which aims to detect, classify, and predict critical failures. This paper presents approaches for constructing a prognostic system.
Introduction
Optimization of maintenance costs is among operators’ main concerns in the search for operational efficiency, safety, and asset availability.
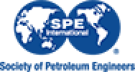