As the major US unconventional basins become more crowded and operators push the limits of well spacing, production impacts from fracture-driven interference between two or more wells, also known as frac hits, are becoming a primary concern.
This has created demand for service companies and technology startups to find ways of diagnosing, predicting, and ultimately mitigating frac hits from taking place during hydraulic fracturing. A race for innovation is under way with a number of possible solutions in the works. One company hoping to stay ahead of the pack on this front is Baker Hughes, a GE company (BHGE).
To BHGE, frac hits are something its operator customers “need an answer for now,” said Hayley Stephenson, reservoir analytics engineer at the company’s Energy Innovation Center in Oklahoma City, Oklahoma. In some fields, child wells, or newer wells, are resulting in just 20–40% of the production of parent wells, or older wells, she observed.
“This is becoming an increasingly big issue for the industry due to the uptrend in child wells being drilled,” Stephenson said, noting that in the Eagle Ford Shale of South Texas, the number of child wells has surpassed the number of parent wells. The question becomes: How do you drill new wells without impacting parent well production or dooming child well production from the outset? The answer isn’t easy because fracture-driven interference is a complicated problem with numerous variables.
BHGE is currently developing an analytics and machine-learning approach that offers descriptive and predictive insights on frac hits, with the aim of eventually offering a real-time monitoring capability to be deployed during frac jobs.
Diagnosing a Hit
During the recent SPE Liquids-Rich Basin Conference in Midland, Texas, Stephenson presented a case study in which the approach provided well spacing rules and completion design recommendations to aid future development in a US onshore basin (SPE 191789). The study team was able to classify the severity of frac hits, and then show through a visualization tool which wells communicated with each other.
BHGE analyzed a 10-well pad with two parent producers and eight child wells after obtaining operator completion data and offset pressure time-series monitoring data. One parent well was on production for about a year, while the other was flowing for around 100 days.
It was noted that seven of the child wells performed worse than the parent wells, with one child well not even reaching 30% of output of either of the parent wells. Meanwhile, a lower rate of production was seen from the second parent well, while both parent wells showed “a huge increase” in water cut and severe pressure depletion, Stephenson said.
“Just looking at these variables at first, we knew that some sort of communication had probably occurred during the completion job,” she said. This was confirmed through offset pressure monitoring, which showed that as frac stages became active, there was pressure response in offset wells. Additionally, oil tracers were recovered in multiple other wells.
The next step was to determine which wells communicated with each other. BHGE utilized information fusion to indicate if a well participated in a communication event. Pressure signatures, oil and water tracer information, and production interference test results were used to produce a single interaction matrix that estimated the likelihood of a well-pair interaction. “Once we were able to see this result,” Stephenson said, “the question then became how specific can we get? Can we get this down to a stage level, and can we do it in a way that can be repeated and isn’t dependent on the data sources, the field, or the user?”
The workflow that BHGE ultimately created consists of two data sets, which are fed into descriptive analysis. The first set, the input data, typically represents parameters of a completion job that were determined before it took place, such as well spacing, pumping schedules, and proppant concentrations. The second set, the output data or “event” data, is what results from the input data—in this case, time-series pressure data on the offset wells. Both data sources are fed into a machine-learning model.
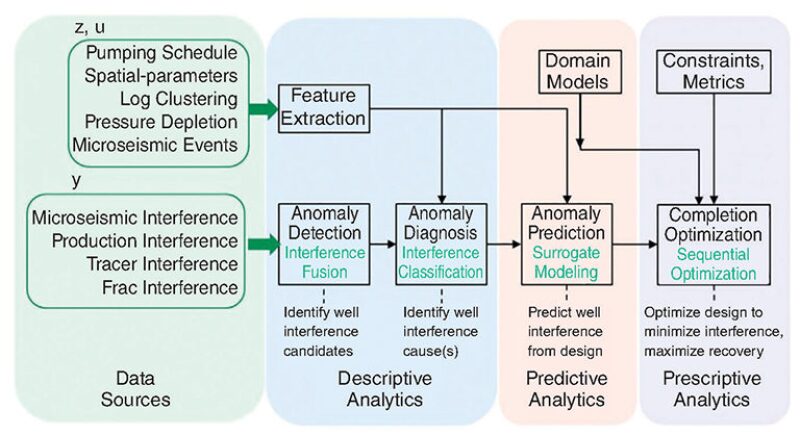
“The machine-learning model recognizes a frac hit occurred, determines what was going on in a stage that might have caused the hit, and then, based on a series of decision trees and clustering analysis, spits out a list of rules, reasons, or decisions for why the hits occur,” she said.
Turning Insight Into Action
For the 10-well pad, more than 14,000 rules and reasons as to why the hits occurred were spit out through the model. A subject matter expert—a veteran engineer—was able to trim that list to 11,000 and then filter the rules through three different categories: simplicity, or the rules with the fewest explainers; coverage, or the rules that were linked to the most frac hits; and efficiency, or how often the rule was correct.
These rules are displayed on an interactive dashboard that provides a 3D, high-level view of the well pad and an overview of the frac hit matrix, showing where the hits occurred and the severity. Here, the user can click on a visual of each of the frac hits and glean individual insights. After BHGE receives data from an operator, it is able to provide the full-functioning visualization tool in a specific timeframe.
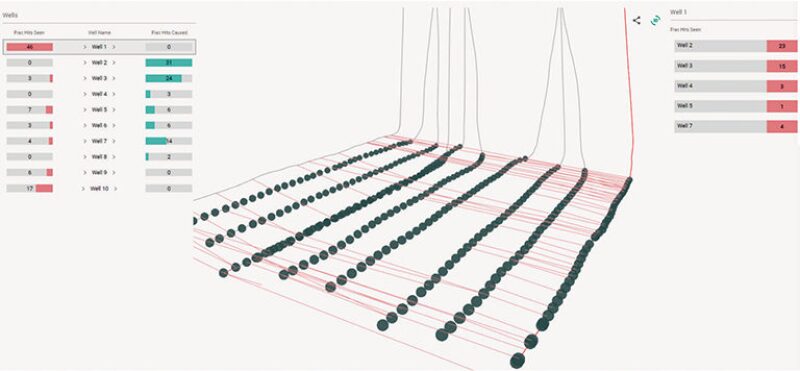
The team is working to build the predictive aspect to a point where it will show when and where a frac hit occurs before it actually occurs. “Because it’s a machine-learning approach, the more data we get, the more certain we are. And the lower the uncertainty, the more we trust our model,” Stephenson said. That will lead to the prescriptive component offering parameters that can be changed to help control or prevent a hit. “This is where our continued development is moving.”
BHGE hopes to create a real-time capability at a latency that allows for immediate action to be taken during frac jobs. “As we move towards the real-time component, we’re looking at updating that insight page after every stage, well, or 24 hours, depending on what the operator wants,” she said.
Stephenson noted that BHGE’s approach is unique in that it doesn’t require additional hardware installation or certain data besides that relating to offset pressure. “With data acquisition being so expensive on well sites, it’s really important that we’re dataset independent. We want to be able to provide answers customizable to the data already being collected … to increase overall operational efficiency.”