In recent years, advancements in artificial intelligence, particularly the revolutionary applications of deep-learning-based computer vision and large language models, have significantly affected the oil and gas industry. These technological developments have underscored the growing importance and diversity of data analytics across various aspects of the industry’s daily operations.
Maintaining this momentum, data analytics practitioners in the industry have reexamined existing workflows and realized the substantial benefits that artificial intelligence brings, including increased efficiency and expedited turnaround times.
Organizations have allocated resources to develop a big-data-driven, multidisciplinary platform that leverages artificial intelligence and machine learning to enhance rapid and robust decision-making in asset management and risk mitigation. Integrated data-management platforms use both proprietary data and public domain data to streamline and automate processes that otherwise could be tedious and time-consuming.
Successful case studies have been shared in the literature on topics such as candidate screening and production forecasting. A prominent area of focus garnering extensive attention is the integration of artificial intelligence and domain knowledge, exemplified by physics-informed machine learning.
Several innovative workflows using proxy or surrogate models in conjunction with machine learning and deep learning have been published. These workflows demonstrate promising test cases that extract valuable insights from physics‑based modeling results and provide production forecasts through a statistical approach. Undoubtedly, we can anticipate a surge in research and publications on this topic in the coming years, which will contribute significantly to reshaping our industry.
As advancements in generative artificial intelligence and big data continue to accelerate, swiftly adapting and pivoting toward digital transformation will be essential in the emerging digital era.
This Month’s Technical Papers
Physics-Informed Deep-Learning Models Improve Forecast Scalability, Reliability
Data-Analytics-Driven Sectorization Review Enables Efficient Reservoir Management
Production-Enhancement-Candidate Screening Powered by ML Unlocks Brownfield Potential
Recommended Additional Reading
SPE 217665 Well Construction in the Era of Big Data: It’s Not Data Analytics, It’s Engineering With Data by G.S. Payette, ExxonMobil, et al.
IPTC 24126 Deep-Learning-Based Long Short-Term Memory for Production Data Analysis of Hydraulically Fractured Wells by Fengyuan Zhang, China University of Petroleum-Beijing, et al.
URTeC 3857064 Application of Data Analytics to Chemometric Analysis of Conventionally Produced Oil Samples From the Delaware Basinby Timothy Carpp, Texas A&M University, et al.
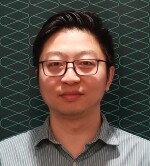
Junjie Yang, SPE, is a senior simulation engineer with Chevron. He has 11 years of experience in the oil and gas industry. Yang’s specialties include analytical and numerical reservoir modeling, hydraulic-fracture modeling, and optimization. He is also skilled in data analytics as well as integrated statistical analysis in production forecasting, rejuvenation-candidate selection, and field development strategy optimization. Yang has been leading, and contributed to, multidisciplinary consulting projects from major conventional and unconventional plays across North America, including superhot rock geothermal asset stimulation and development. He holds a PhD degree in petroleum and natural gas engineering from The Pennsylvania State University.