The value of hidden-danger data stored in text can be revealed through an approach that can help sort and interpret information in an ordered way not used previously in safety management. These optimized data then can be used to apply safety-management techniques precisely, centralize operations, and reduce risk levels.
Introduction
The collection and storage of huge amounts of data have demonstrated a lack of coordination between the development of data-collecting capacity and the means to analyze those data accurately. Some experts use analytical methods to study the relationship between security factors and accident events to guide equipment maintenance, quality testing, and related work. However, extracting hidden-danger data stored in text format has been a challenge for the petroleum and petrochemical industries.
The data-mining technique discussed in the complete paper uses Chinese word segmentation, Chinese lexical annotation, named entity recognition, and other techniques to extract keywords from the text.
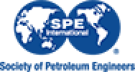