This study presents an efficient gradient-based production optimization method that uses a deep-learning-based proxy model for the prediction of state variables (such as pressures and saturations) and well outputs [such as bottomhole pressures (BHPs) and injection rates] to solve nonlinearly constrained optimization with geological uncertainty. The surrogate model is the embed-to-control observe (E2CO) deep-learning proxy model, consisting of four blocks of neural networks: encoder, transition, transition output, and decoder.
Background and Problem Statement
Life-cycle production optimization is a crucial step in closed-loop reservoir management (CLRM). Even though numerous problems of interest exist, the specific application considered here refers to the optimization of the well-control problem that maximizes the net present value (NPV), given an ensemble of reservoir models representing the geological uncertainty, as a result of involving various data sources such as geology, seismic data, well logs, well tests, and—most commonly and most importantly—the nonunique nature of the history-matching (or data-assimilation) process. This optimization problem is known as a robust production-optimization problem, and it is even significantly more challenging when nonlinear state constraints are present at each control step and must be honored.
Typically, in traditional CLRM, the evaluation of the objective function and constraint values relies on the outputs from a high-fidelity reservoir simulator (HFS). However, using HFS, whether developed in-house or commercially, for production optimization may impose significant computational demands.
A possible way forward in reducing the computational costs associated with forward simulations is the use of reduced-order models (ROMs). These methods work with a reduced dimension of system states based on the full form of the governing equations, thus enabling sequential prediction of the states’ evolution that could closely represent the true physics. Proper orthogonal decomposition-trajectory piecewise linearization (POD-TPWL) is a commonly used ROM for subsurface flow applications.
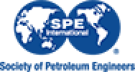